Designing Business Intelligence Dashboards to Support Decision-Making in a Fishery Business
DOI:
https://doi.org/10.12928/biste.v5i4.9207Keywords:
Business Intelligence, Fishery Business, Support Decision-MakingAbstract
Accurate assessment and thorough analysis of managerial performance are essential in obtaining enhanced business performance. A real-time monitoring system is necessary to support the decision-making process. This study aims to design a business intelligence dashboard containing real-time monitoring of water quality to support the decision-making of the management team of an agribusiness company. Four steps were used in designing the business intelligence (BI) dashboard: (1) scope and plan, (2) analyze and define, (3) architect and design, and (4) build, test, and refine. The study started with determining the scope and plan for developing the BI dashboard to monitor the water pond’s quality in real time. The requirements of system input and output were identified in the analyze and define phase. The data warehouse model and design visualization regarding the BI dashboard were determined in the architect and design step. The system's architecture was analyzed in the final step, build and test. Three months of data collection and interviews with the management team of the fishery company were performed to support each step in BI design. This study’s outcome is a BI dashboard providing real-time monitoring that supports the management team's decision-making process. This study still considers two water quality measures; therefore, future research can be conducted using other measures. Future research can also be performed on another agribusiness company to support the decision-making process and increase competitiveness.
References
S. S. Kamble, A. Gunasekaran, A. Ghadge, and R. Raut, “A performance measurement system for industry 4.0 enabled smart manufacturing system in SMMEs-A review and empirical investigation,” International journal of production economics, vol. 229, p. 107853, 2020, https://doi.org/10.1016/j.ijpe.2020.107853.
Tavera Romero, C. A., Ortiz, J. H., Khalaf, O. I., & Ríos Prado, A. (2021). Business intelligence: business evolution after industry 4.0. Sustainability, 13(18), 10026, 2021, https://doi.org/10.3390/su131810026.
C. Burnay, F. Dargam dan P. Zarate, “Data Visualization for Decision-Making: An Important Issue,” Operational Research, vol. 19, no. 4, pp. 174-190, 2020, https://doi.org/10.1007/s12351-019-00530-z.
W. C. Hsu, P. Y. Chao, C. S. Wang, J. C. Hsieh, and W. Huang, “Application of regression analysis to achieve a smart monitoring system for aquaculture,” Information, vol. 11, no. 8, p. 387, 2020, https://doi.org/10.3390/info11080387.
N. Ain, G. Vaia, W. H. DeLone, and M. Waheed, “Two decades of research on business intelligence system adoption, utilization and success–A systematic literature review,” Decision Support Systems, vol. 125, p. 113113, 2019, https://doi.org/10.1016/j.dss.2019.113113.
F. E. Bordeleau, E. Mosconi, and L. A. de Santa-Eulalia, “Business intelligence and analytics value creation in Industry 4.0: a multiple case study in manufacturing medium enterprises,” Production Planning & Control, vol. 31, no. 2-3, pp. 173-185, 2020, https://doi.org/10.1080/09537287.2019.1631458.
O. Moscoso-Zea, J. Castro, J. Paredes-Gualtor and S. Luján-Mora, "A Hybrid Infrastructure of Enterprise Architecture and Business Intelligence & Analytics for Knowledge Management in Education," in IEEE Access, vol. 7, pp. 38778-38788, 2019, https://doi.org/10.1109/ACCESS.2019.2906343.
W. A. Jabbar et al., "Design and Fabrication of Smart Home With Internet of Things Enabled Automation System," in IEEE Access, vol. 7, pp. 144059-144074, 2019, https://doi.org/10.1109/ACCESS.2019.2942846.
S. Croce and S. Tondini, “Fixed and Mobile Low-Cost Sensing Approaches for Microclimate Monitoring in Urban Areas: A Preliminary Study in the City of Bolzano (Italy),” Smart Cities, vol. 5, no. 1, pp. 54-70, 2022, https://doi.org/10.3390/smartcities5010004.
B. Nour, S. Cherkaoui and Z. Mlika, "Federated Learning and Proactive Computation Reuse at the Edge of Smart Homes," in IEEE Transactions on Network Science and Engineering, vol. 9, no. 5, pp. 3045-3056, 1 Sept.-Oct. 2022, https://doi.org/10.1109/TNSE.2021.3131246.
Y. Cardinale, G. Freites, E. Valderrama, A. Aguilera, and C. Angsuchotmetee, “Semantic framework of event detection in emergency situations for smart buildings,” Digital Communications and Networks, vol. 8, no. 1, pp. 64-79, 2022, https://doi.org/10.1016/j.dcan.2021.06.005.
Y. K. Kalkhajeh, B. Huang, W. Hu, C. Ma, H. Gao, M. L. Thompson, and H. C. B. Hansen, “Environmental soil quality and vegetable safety under current greenhouse vegetable production management in China,” Agriculture, Ecosystems & Environment, vol. 307, p. 107230, 2021, https://doi.org/10.1016/j.agee.2020.107230.
K. T. Kadhim, A. M. Alsahlany, S. M. Wadi, and H. T. Kadhum, “An overview of patient’s health status monitoring system based on internet of things (IoT),” Wireless Personal Communications, vol. 114, no. 3, pp. 2235-2262, 2020, https://doi.org/10.1007/s11277-020-07474-0.
J. Ranjan and C. Foropon, “Big data analytics in building the competitive intelligence of organizations,” International Journal of Information Management, vol. 56, p. 102231, 2021, https://doi.org/10.1016/j.ijinfomgt.2020.102231.
G. Richards, W. Yeoh, A. Y. L. Chong, and A. Popovič, “Business intelligence effectiveness and corporate performance management: an empirical analysis,” Journal of Computer Information Systems, vol. 59, no. 2, pp. 188-196, 2019, https://doi.org/10.1080/08874417.2017.1334244.
G. Kamulegeya, R. Mugwanya and R. Hebig, "Requirements for Measurement Dashboards and Their Benefits: A Study of Start-ups in an Emerging Ecosystem," 2019 45th Euromicro Conference on Software Engineering and Advanced Applications (SEAA), pp. 300-308, 2019, https://doi.org/10.1109/SEAA.2019.00053.
E. B. Hammond, F. Coulon, S. H. Hallett, R. Thomas, D. Hardy, A. Kingdon, and D. J. Beriro, “A critical review of decision support systems for brownfield redevelopment,” Science of the Total Environment, vol. 785, p. 147132, 2021, https://doi.org/10.1016/j.scitotenv.2021.147132.
P. Tambare, C. Meshram, C. C. Lee, R. J. Ramteke, and A. L. Imoize, “Performance measurement system and quality management in data-driven Industry 4.0: A review,” Sensors, vol. 22, no. 1, p. 224, 2021, https://doi.org/10.3390/s22010224.
F. Jan, N. Min-Allah, and D. Düştegör, “Iot based smart water quality monitoring: Recent techniques, trends and challenges for domestic applications,” Water, vol. 13, no. 13, p. 1729, 2021, https://doi.org/10.3390/w13131729.
S. Ragaveena, A. Shirly Edward, and U. Surendran, ‘Smart controlled environment agriculture methods: A holistic review,” Reviews in Environmental Science and Bio/Technology, vol. 20, no. 4, pp. 887-913, 2021, https://doi.org/10.1007/s11157-021-09591-z.
N. Mahfuz and S. M. Al-Mayeed, "Smart Monitoring and Controlling System for Aquaculture of Bangladesh to Enhance Robust Operation," 2020 IEEE Region 10 Symposium (TENSYMP), Dhaka, Bangladesh, 2020, pp. 1128-1133, 2020, https://doi.org/10.1109/TENSYMP50017.2020.9230748.
Y. -B. Lin and H. -C. Tseng, "FishTalk: An IoT-Based Mini Aquarium System," in IEEE Access, vol. 7, pp. 35457-35469, 2019, https://doi.org/10.1109/ACCESS.2019.2905017.
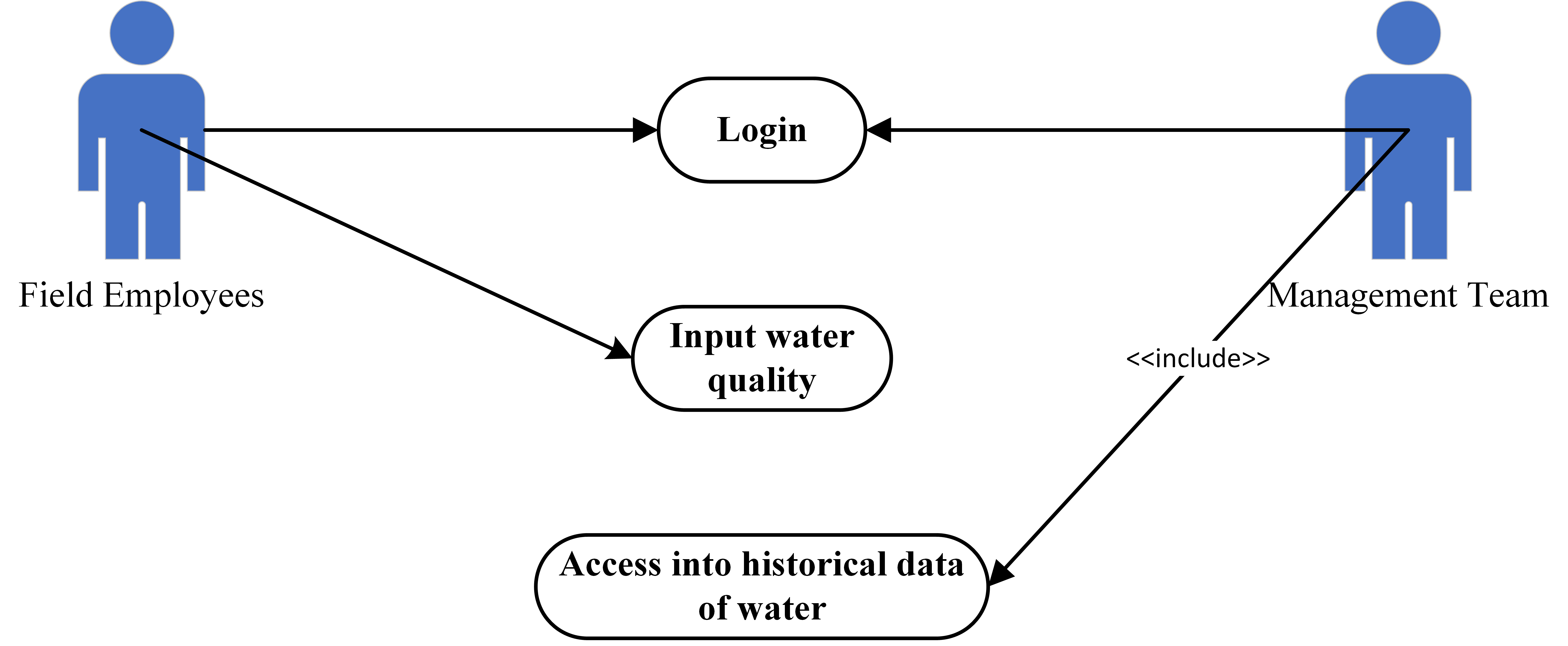
Downloads
Published
How to Cite
Issue
Section
License
Copyright (c) 2023 Muhammad Satya Raihanto, Melinska Ayu Febrianti, Qurtubi, Danang Setiawan, Windi Auliana

This work is licensed under a Creative Commons Attribution-ShareAlike 4.0 International License.
Authors who publish with this journal agree to the following terms:
- Authors retain copyright and grant the journal right of first publication with the work simultaneously licensed under a Creative Commons Attribution License that allows others to share the work with an acknowledgment of the work's authorship and initial publication in this journal.
- Authors are able to enter into separate, additional contractual arrangements for the non-exclusive distribution of the journal's published version of the work (e.g., post it to an institutional repository or publish it in a book), with an acknowledgment of its initial publication in this journal.
- Authors are permitted and encouraged to post their work online (e.g., in institutional repositories or on their website) prior to and during the submission process, as it can lead to productive exchanges, as well as earlier and greater citation of published work (See The Effect of Open Access).
This journal is licensed under a Creative Commons Attribution-ShareAlike 4.0 International License.