Bibliometric Analysis of Explainable AI in Advance Care Planning: Insights, Collaborative Trends, and Future Prospects
DOI:
https://doi.org/10.12928/biste.v6i4.11641Keywords:
Explainable AI, Advance Care Planning, Bibliometric Analysis, Healthcare Analytics, Decision Support SystemsAbstract
The increasing complexity of healthcare systems has led to a growing need for Advance Care Planning (ACP) to ensure personalized care for patients. Explainable Artificial Intelligence (XAI) has emerged as a promising solution to enhance ACP by providing transparent and interpretable decision-making processes. However, the current landscape of XAI in ACP remains unclear, necessitating a comprehensive bibliometric analysis. This study employed a systematic review of existing literature on XAI in ACP, using a bibliometric approach to analyze publication trends, collaboration patterns, and research themes. One hundred sixty articles were selected from prominent databases, and their metadata were extracted and analyzed using Biblioshiny, the analysis revealed a significant growth in ACP XAI-related publications, focusing on deep learning and natural language processing techniques. The top contributing authors and institutions were identified, and their collaborative networks were visualized. The results also highlighted the prominent themes of patient-centered care, decision support systems, and healthcare analytics. The study's findings have implications for developing more effective XAI-based ACP systems. This bibliometric analysis provides valuable insights into the current state of XAI in ACP, highlighting the need for further research and collaboration to address the complex challenges in healthcare. The study's outcomes can inform policymakers, researchers, and practitioners in developing more effective ACP systems that leverage the potential of XAI.
References
WHO, ‘Ageing and health’. Accessed: Oct. 23, 2024. https://www.who.int/news-room/fact-sheets/detail/ageing-and-health.
A. Balachandran, “Population ageing in Europe and Asia: Beyond traditional perspectives,” University of Groningen, 2020, https://doi.org/10.33612/diss.135497884.
G. Shackman, X. Wang, and Y.-L. Liu, “Brief Review of World Demographic Trends - Trends in Age Distributions,” SSRN Electron. J., 2012, https://doi.org/10.2139/ssrn.2180600.
B. C. Vladeck and J. P. Firman, “The Aging of the Population and Health Services,” Ann. Am. Acad. Pol. Soc. Sci., vol. 468, no. 1, pp. 132–148, 1983, https://doi.org/10.1177/0002716283468001009.
F. T. Denton and B. G. Spencer, “Chronic Health Conditions: Changing Prevalence in an Aging Population and Some Implications for the Delivery of Health Care Services,” Can. J. Aging Rev. Can. Vieil., vol. 29, no. 1, pp. 11–21, 2010, https://doi.org/10.1017/S0714980809990390.
T. M. Dall, P. D. Gallo, R. Chakrabarti, T. West, A. P. Semilla, and M. V. Storm, “An Aging Population And Growing Disease Burden Will Require ALarge And Specialized Health Care Workforce By 2025,” Health Aff. (Millwood), vol. 32, no. 11, pp. 2013–2020, 2013, https://doi.org/10.1377/hlthaff.2013.0714.
T. R. Fried, E. H. Bradley, V. R. Towle, and H. Allore, “Understanding the Treatment Preferences of Seriously Ill Patients,” N. Engl. J. Med., vol. 346, no. 14, pp. 1061–1066, 2002, https://doi.org/10.1056/NEJMsa012528.
J. S. Weiner and S. A. Cole, “Three Principles to Improve Clinician Communication for Advance Care Planning: Overcoming Emotional, Cognitive, and Skill Barriers,” J. Palliat. Med., vol. 7, no. 6, pp. 817–829, 2004, https://doi.org/10.1089/jpm.2004.7.817.
M.-K. Song et al., “Effects of an intervention to improve communication about end-of-life care among African Americans with chronic kidney disease,” Appl. Nurs. Res., vol. 23, no. 2, pp. 65–72, 2010, https://doi.org/10.1016/j.apnr.2008.05.002.
J. L. Holley and J. A. Yasunaga, “Advance Care Planning to Elicit and Respect Patient Values and Preferences,” in Palliative Care in Nephrology, pp. 98–107, 2020, https://doi.org/10.1093/med/9780190945527.003.0010.
W. E. Rosa et al., “Advance Care Planning in Serious Illness: A Narrative Review,” J. Pain Symptom Manage., vol. 65, no. 1, pp. e63–e78, 2023, https://doi.org/10.1016/j.jpainsymman.2022.08.012.
R. C. Kolarik, R. M. Arnold, G. S. Fischer, and J. A. Tulsky, “Objectives for Advance Care Planning,” J. Palliat. Med., vol. 5, no. 5, pp. 697–704, 2002, https://doi.org/10.1089/109662102320880516.
C. Sedini, M. Biotto, L. M. Crespi Bel’skij, R. E. Moroni Grandini, and M. Cesari, “Advance care planning and advance directives: an overview of the main critical issues,” Aging Clin. Exp. Res., vol. 34, no. 2, pp. 325–330, 2022, https://doi.org/10.1007/s40520-021-02001-y.
M. Butler, E. Ratner, E. McCreedy, N. Shippee, and R. L. Kane, “Decision Aids for Advance Care Planning: An Overview of the State of the Science”, Ann. Intern. Med., vol. 161, no. 6, p. 408, 2014, https://doi.org/10.7326/M14-0644.
C. E. Low et al., “A cross-sectional study on advance care planning documentation attitudes during national advance care planning week in a South-East Asian country,” BMC Palliat. Care, vol. 23, no. 1, p. 244, 2024, https://doi.org/10.1186/s12904-024-01505-4.
C. Burris and D. Sevier, “Sidebar: Enhancing Technology to Better Communicate Advance Care Planning Information,” N. C. Med. J., vol. 81, no. 4, pp. 245–246, 2020, https://doi.org/10.18043/ncm.81.4.245.
M. J. Green and B. H. Levi, ‘The era of “e”: The use of new technologies in advance care planning’, Nurs. Outlook, vol. 60, no. 6, pp. 376-383.e2, 2012, https://doi.org/10.1016/j.outlook.2012.08.005.
S. Bose-Brill and T. R. Pressler, “Commentary: Opportunities for Innovation and Improvement in Advance Care Planning Using a Tethered Patient Portal in the Electronic Health Record,” J. Prim. Care Community Health, vol. 3, no. 4, pp. 285–288, 2012, https://doi.org/10.1177/2150131911435525.
J. Kraft, “Injecting social technology into advance care planning,” BMJ Support. Palliat. Care, vol. 2, no. 2, p. 199.2-199, 2012, https://doi.org/10.1136/bmjspcare-2012-000250.107.
T. Davenport and R. Kalakota, “The potential for artificial intelligence in healthcare,” Future Healthc. J., vol. 6, no. 2, pp. 94–98, 2019, https://doi.org/10.7861/futurehosp.6-2-94.
L. De Panfilis, C. Peruselli, S. Tanzi, and C. Botrugno, “AI-based clinical decision-making systems in palliative medicine: ethical challenges,” BMJ Support. Palliat. Care, vol. 13, no. 2, pp. 183–189, 2023, https://doi.org/10.1136/bmjspcare-2021-002948.
D. C. McElfresh et al., “Can an Algorithm Be My Healthcare Proxy?,” in Explainable AI in Healthcare and Medicine, vol. 914, pp. 313–319, 2021, https://doi.org/10.1007/978-3-030-53352-6_30.
C. Giordano, M. Brennan, B. Mohamed, P. Rashidi, F. Modave, and P. Tighe, “Accessing Artificial Intelligence for Clinical Decision-Making,” Front. Digit. Health, vol. 3, p. 645232, 2021, https://doi.org/10.3389/fdgth.2021.645232.
G. Cano-Verdugo, J. Owoyemi, A. Khan, M. Sadman Tahsin, N. Jiwani, and D. N. Shukurullaevna, “Leveraging Artificial Intelligence to Revolutionize Healthcare: A Comprehensive Analysis,” SciWaveBulletin, vol. 01, no. 01, pp. 12–19, 2023, https://doi.org/10.61925/SWB.2023.1103.
M. Ghassemi, L. Oakden-Rayner, and A. L. Beam, “The false hope of current approaches to explainable artificial intelligence in health care,” Lancet Digit. Health, vol. 3, no. 11, pp. e745–e750, 2021, https://doi.org/10.1016/S2589-7500(21)00208-9.
A. F. Markus, J. A. Kors, and P. R. Rijnbeek, “The role of explainability in creating trustworthy artificial intelligence for health care: A comprehensive survey of the terminology, design choices, and evaluation strategies,” J. Biomed. Inform., vol. 113, p. 103655, 2021, https://doi.org/10.1016/j.jbi.2020.103655.
N. Bienefeld et al., “Solving the explainable AI conundrum by bridging clinicians’ needs and developers’ goals,” Npj Digit. Med., vol. 6, no. 1, p. 94, 2023, https://doi.org/10.1038/s41746-023-00837-4.
C. C. Yang, “Explainable Artificial Intelligence for Predictive Modeling in Healthcare,” J. Healthc. Inform. Res., vol. 6, no. 2, pp. 228–239, 2022, https://doi.org/10.1007/s41666-022-00114-1.
N. Rane, S. Choudhary, and J. Rane, “Explainable Artificial Intelligence (XAI) in healthcare: Interpretable Models for Clinical Decision Support,” SSRN Electron. J., 2023, https://doi.org/10.2139/ssrn.4637897.
R. Mariappan, “Extensive Review of Literature on Explainable AI (XAI) in HealthcareApplications’, Recent Adv. Comput. Sci. Commun., vol. 17, 2024, https://doi.org/10.2174/0126662558296699240314055348.
Z. Sadeghi et al., “A brief review of explainable artificial intelligence in healthcare,” arXiv preprint arXiv:2304.01543, 2023, https://doi.org/10.48550/arXiv.2304.01543.
T. Hulsen, “Explainable Artificial Intelligence (XAI): Concepts and Challenges in Healthcare,” AI, vol. 4, no. 3, pp. 652–666, 2023, https://doi.org/10.3390/ai4030034.
U. B. Khakurel and D. B. Rawat, “Evaluating explainable artificial intelligence (XAI): algorithmic explanations for transparency and trustworthiness of ML algorithms and AI systems,” in Artificial Intelligence and Machine Learning for Multi-Domain Operations Applications IV, p. 7, 2022, https://doi.org/10.1117/12.2620598.
N. Thalpage, “Unlocking the Black Box: Explainable Artificial Intelligence (XAI) for Trust and Transparency in AI Systems,” J. Digit. Art Humanit., vol. 4, no. 1, pp. 31–36, 2023, https://doi.org/10.33847/2712-8148.4.1_4.
R. Tiwari, “Explainable AI (XAI) and its Applications in Building Trust and Understanding in AI Decision Making,” INTERANTIONAL J. Sci. Res. Eng. Manag., vol. 07, no. 01, 2023, https://doi.org/10.55041/IJSREM17592.
R. S. Deshpande, P. V. Ambatkar, “Interpretable Deep Learning Models: Enhancing Transparency and Trustworthiness in Explainable AI,” Proceeding Int. Conf. Sci. Eng., vol. 11, no. 1, pp. 1352–1363, 2023, https://doi.org/10.52783/cienceng.v11i1.286.
Q. Xu et al., “Interpretability of Clinical Decision Support Systems Based on Artificial Intelligence from Technological and Medical Perspective: A Systematic Review,” J. Healthc. Eng., vol. 2023, no. 1, p. 9919269, 2023, https://doi.org/10.1155/2023/9919269.
T. Sethi, A. Kalia, A. Sharma, and A. Nagori, “Interpretable artificial intelligence: Closing the adoption gap in healthcare,” in Artificial Intelligence in Precision Health, pp. 3–29, 2020, https://doi.org/10.1016/B978-0-12-817133-2.00001-X.
A. Duenser and D. M. Douglas, “Whom to Trust, How and Why: Untangling Artificial Intelligence Ethics Principles, Trustworthiness, and Trust,” IEEE Intell. Syst., vol. 38, no. 6, pp. 19–26, 2023, https://doi.org/10.1109/MIS.2023.3322586.
J. Zhang and Z. Zhang, “Ethics and governance of trustworthy medical artificial intelligence,” BMC Med. Inform. Decis. Mak., vol. 23, no. 1, p. 7, 2023, https://doi.org/10.1186/s12911-023-02103-9.
R. Dlugatch, A. Georgieva, and A. Kerasidou, “Trustworthy artificial intelligence and ethical design: public perceptions of trustworthiness of an AI-based decision-support tool in the context of intrapartum care,” BMC Med. Ethics, vol. 24, no. 1, p. 42, 2023, https://doi.org/10.1186/s12910-023-00917-w.
C. González-Gonzalo et al., “Trustworthy AI: Closing the gap between development and integration of AI systems in ophthalmic practice,” Prog. Retin. Eye Res., vol. 90, p. 101034, 2022, https://doi.org/10.1016/j.preteyeres.2021.101034.
H. D. Lum, R. L. Sudore, and D. B. Bekelman, “Advance Care Planning in the Elderly,” Med. Clin. North Am., vol. 99, no. 2, pp. 391–403, 2015, https://doi.org/10.1016/j.mcna.2014.11.010.
L. E. Dingfield and J. B. Kayser, “Integrating Advance Care Planning Into Practice,” Chest, vol. 151, no. 6, pp. 1387–1393, 2017, https://doi.org/10.1016/j.chest.2017.02.024.
N. Pease, “Advance care-planning and clinical decision-making,” Medicine (Baltimore), vol. 48, no. 1, pp. 57–60, 2020, https://doi.org/10.1016/j.mpmed.2019.10.012.
S. S. Sullivan, “TakingAIM: A Precision Health Framework for Promoting Person-Centered Advance Care Planning,” J. Hosp. Palliat. Nurs., vol. 21, no. 6, pp. 502–509, 2019, https://doi.org/10.1097/NJH.0000000000000560.
A. Choudhury, “Toward an Ecologically Valid Conceptual Framework for the Use of Artificial Intelligence in Clinical Settings: Need for Systems Thinking, Accountability, Decision-making, Trust, and Patient Safety Considerations in Safeguarding the Technology and Clinicians,” JMIR Hum. Factors, vol. 9, no. 2, p. e35421, 2022, https://doi.org/10.2196/35421.
I. Passas, “Bibliometric Analysis: The Main Steps,” Encyclopedia, vol. 4, no. 2, pp. 1014–1025, 2024, https://doi.org/10.3390/encyclopedia4020065.
D. F. Thompson and C. K. Walker, “A Descriptive and Historical Review of Bibliometrics with Applications to Medical Sciences,” Pharmacother. J. Hum. Pharmacol. Drug Ther., vol. 35, no. 6, pp. 551–559, 2015, https://doi.org/10.1002/phar.1586.
M. D. S. T. Silva, V. M. D. Oliveira, and S. É. N. Correia, “Scientific mapping in Scopus with Biblioshiny: A bibliometric analysis of organizational tensions,” Context. – Rev. Contemp. Econ. E Gest., vol. 20, pp. 54–71, 2022, https://doi.org/10.19094/contextus.2022.72151.
H. K. Kondaveeti, S. Raman, and P. Raj, “Prototyping with Raspberry Pi in Healthcare Domain,” Int. J. Adv. Comput. Sci. Appl., vol. 11, no. 10, 2020, https://doi.org/10.14569/IJACSA.2020.0111038.
M. N. Akhtar, A. Haleem, and M. Javaid, “Exploring the advent of Medical 4.0: A bibliometric analysis systematic review and technology adoption insights,” Inform. Health, vol. 1, no. 1, pp. 16–28, 2024, https://doi.org/10.1016/j.infoh.2023.10.001.
A. A. Olariu, A. Breazu, Ștefan C. Popa, C. F. Popa, and N. Căruceru, “Decision-Making in Healthcare. A Bibliometric Exploration,” Proc. Int. Conf. Bus. Excell., vol. 18, no. 1, pp. 3242–3254, 2024, https://doi.org/10.2478/picbe-2024-0264.
E. M. Kenny, E. D. Delaney, D. Greene, and M. T. Keane, “Post-hoc Explanation Options for XAI in Deep Learning: The Insight Centre for Data Analytics Perspective,” in Pattern Recognition. ICPR International Workshops and Challenges, vol. 12663, pp. 20–34, 2021, https://doi.org/10.1007/978-3-030-68796-0_2.
G. Yang, Q. Ye, and J. Xia, “Unbox the black-box for the medical explainable AI via multi-modal and multi-centre data fusion: A mini-review, two showcases and beyond,” Inf. Fusion, vol. 77, pp. 29–52, 2022, https://doi.org/10.1016/j.inffus.2021.07.016.
D. Wang, Q. Yang, A. Abdul, and B. Y. Lim, “Designing Theory-Driven User-Centric Explainable AI,” in Proceedings of the 2019 CHI Conference on Human Factors in Computing Systems, , pp. 1–15, 2019, https://doi.org/10.1145/3290605.3300831.
B. L. Kass-Bartelmes and R. Hughes, “Advance Care Planning: Preferences for Care at the End of Life,” J. Pain Palliat. Care Pharmacother., vol. 18, no. 1, pp. 87–109, 2004, https://doi.org/10.1080/J354v18n01_08.
A. U. Patel, Q. Gu, R. Esper, D. Maeser, and N. Maeser, “The Crucial Role of Interdisciplinary Conferences in Advancing Explainable AI in Healthcare,” BioMedInformatics, vol. 4, no. 2, pp. 1363–1383, 2024, https://doi.org/10.3390/biomedinformatics4020075.
S. Ali, A. S. Imran, Z. Kastrati, and S. M. Daudpota, “Visualizing Research on Explainable Artificial Intelligence for Medical and Healthcare,” in 2023 4th International Conference on Computing, Mathematics and Engineering Technologies (iCoMET), pp. 1–6, 2023, https://doi.org/10.1109/iCoMET57998.2023.10099343.
C.-J. Liu et al., “A Worldwide Bibliometric Analysis of Publications on Advance Care Planning in the Past 3 Decades,” Am. J. Hosp. Palliat. Med., vol. 37, no. 6, pp. 474–480, 2020, https://doi.org/10.1177/1049909119886305.
Y. Lai, A. Kankanhalli, and D. Ong, “Human-AI Collaboration in Healthcare: A Review and Research Agenda,” presented at the Hawaii International Conference on System Sciences, 2021, https://doi.org/10.24251/HICSS.2021.046.
J. Baas, M. Schotten, A. Plume, G. Côté, and R. Karimi, “Scopus as a curated, high-quality bibliometric data source for academic research in quantitative science studies,” Quant. Sci. Stud., vol. 1, no. 1, pp. 377–386, 2020, https://doi.org/10.1162/qss_a_00019.
B. L. Jimma, “Artificial intelligence in healthcare: A bibliometric analysis,” Telemat. Inform. Rep., vol. 9, p. 100041, 2023, https://doi.org/10.1016/j.teler.2023.100041.
M. Schotten, M. El Aisati, W. J. N. Meester, S. Steiginga, and C. A. Ross, “A Brief History of Scopus: The World’s Largest Abstract and Citation Database of Scientific Literature,” in Research Analytics, pp. 31–58, 2017, https://doi.org/10.1201/9781315155890-3.
M. Visser, N. J. Van Eck, and L. Waltman, “Large-scale comparison of bibliographic data sources: Scopus, Web of Science, Dimensions, Crossref, and Microsoft Academic,” Quant. Sci. Stud., vol. 2, no. 1, pp. 20–41, 2021, https://doi.org/10.1162/qss_a_00112.
V. Devaki, Dr. E. Ramganesh, and Dr. S. Amutha, “Bibliometric Analysis on Metacognition and Self-Regulation Using Biblioshiny Software,” Indian J. Inf. Sources Serv., vol. 14, no. 2, pp. 115–125, 2024, https://doi.org/10.51983/ijiss-2024.14.2.17 .
J. Ali, A. Jusoh, N. Idris, A. G. Airij, and R. Chandio, “Wearable Devices in Healthcare Services. Bibliometrix Analysis by using R Package,” Int. J. Online Biomed. Eng. IJOE, vol. 18, no. 08, pp. 61–86, 2022, https://doi.org/10.3991/ijoe.v18i08.31785.
C. R. Manz et al., “Validation of a Machine Learning Algorithm to Predict 180-Day Mortality for Outpatients With Cancer,” JAMA Oncol., vol. 6, no. 11, p. 1723, 2020, https://doi.org/10.1001/jamaoncol.2020.4331.
K. Jung et al., “A framework for making predictive models useful in practice,” J. Am. Med. Inform. Assoc., vol. 28, no. 6, pp. 1149–1158, 2021, https://doi.org/10.1093/jamia/ocaa318.
E. Vu et al., “Applications of Machine Learning in Palliative Care: A Systematic Review,” Cancers, vol. 15, no. 5, p. 1596, 2023, https://doi.org/10.3390/cancers15051596.
M. Knop, S. Weber, M. Mueller, and B. Niehaves, “Human Factors and Technological Characteristics Influencing the Interaction of Medical Professionals With Artificial Intelligence–Enabled Clinical Decision Support Systems: Literature Review,” JMIR Hum. Factors, vol. 9, no. 1, p. e28639, 2022, https://doi.org/10.2196/28639.
I. Dankwa-Mullan, “Health Equity and Ethical Considerations in Using Artificial Intelligence in Public Health and Medicine,” Prev. Chronic. Dis., vol. 21, p. 240245, 2024, https://doi.org/10.5888/pcd21.240245.
U. Munive Jesus, “Incorporating artificial intelligence in palliative care: opportunities and challenges,” Hosp. Palliat. Med. Int. J., vol. 7, no. 3, pp. 81–83, 2024, https://doi.org/10.15406/hpmij.2024.07.00244.
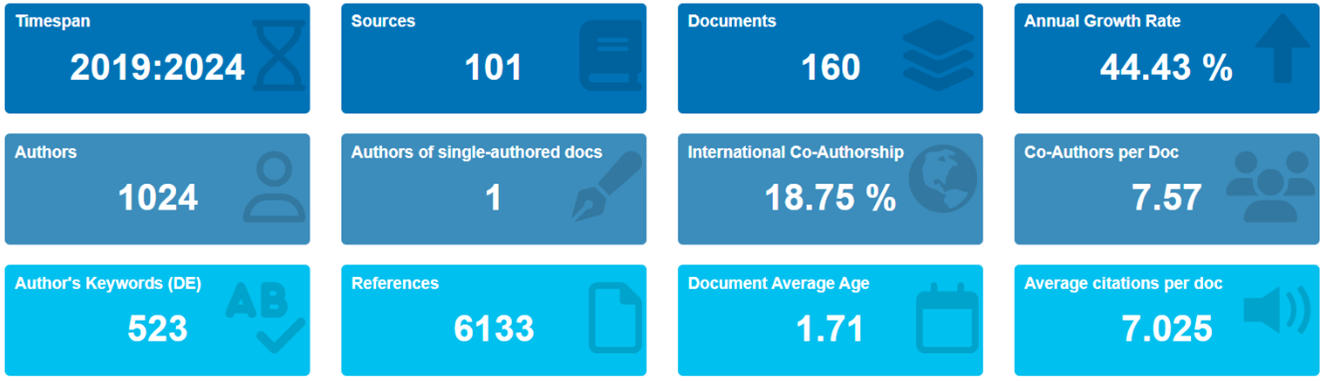
Published
How to Cite
Issue
Section
License
Copyright (c) 2024 Irianna Futri, Elvaro Islami Muryadi, Dimas Chaerul Ekty Saputra

This work is licensed under a Creative Commons Attribution-ShareAlike 4.0 International License.
Authors who publish with this journal agree to the following terms:
- Authors retain copyright and grant the journal right of first publication with the work simultaneously licensed under a Creative Commons Attribution License that allows others to share the work with an acknowledgment of the work's authorship and initial publication in this journal.
- Authors are able to enter into separate, additional contractual arrangements for the non-exclusive distribution of the journal's published version of the work (e.g., post it to an institutional repository or publish it in a book), with an acknowledgment of its initial publication in this journal.
- Authors are permitted and encouraged to post their work online (e.g., in institutional repositories or on their website) prior to and during the submission process, as it can lead to productive exchanges, as well as earlier and greater citation of published work (See The Effect of Open Access).
This journal is licensed under a Creative Commons Attribution-ShareAlike 4.0 International License.