Assessing the Efficacy of the UV Index in Predicting Surface UV Radiation: A Comprehensive Analysis Using Statistical and Machine Learning Methods
DOI:
https://doi.org/10.12928/irip.v6i2.8216Keywords:
UV Index prediction, Public health implications, UV radiation modelling, Predictive analytics, UV protection strategiesAbstract
The study investigated the relationship between the UV Index and measurements of ultraviolet A (UVA) and ultraviolet B (UVB) radiation to evaluate the effectiveness of the UV Index in predicting and understanding UV radiation at the surface. The implications of this study are significant for public health policies and UV protection strategies. This study used a variety of statistical analyses and modelling techniques, including ANOVA, Naive Bayes classification, decision trees, artificial neural networks, support vector machines (SVM), and k-means clustering, to examine relationships and predict UV Index values. ANOVA analysis showed a significant relationship between the UV Index and UVA and UVB measurements. Prediction models such as Naive Bayes classification, decision trees, and artificial neural networks showed variability in their accuracy. Notably, SVM showed a high degree of accuracy in predicting UV Index values, while k-means clustering effectively clustered the data based on similarities in UV Index and UV measurements. These findings confirm that the UV Index is a reliable indicator for predicting and understanding UV radiation levels at the Earth's surface. This research underscores the importance of developing more accurate and precise UV Index prediction models. Further investigation is essential to understand the temporal variations and environmental impacts on the UV Index, as well as the broader implications of UV exposure on public health. This study lays a strong foundation for the development of early warning systems and more effective UV protection strategies, ultimately improving public health outcomes and safety measures against UV radiation.
References
G. H. Bernhard et al., “Environmental effects of stratospheric ozone depletion, UV radiation and interactions with climate change: UNEP Environmental Effects Assessment Panel, update 2019,” Photochem. Photobiol. Sci., vol. 19, no. 5, pp. 542–584, May 2020, https://doi.org/10.1039/d0pp90011g.
S. A. Umar and S. A. Tasduq, “Ozone Layer Depletion and Emerging Public Health Concerns - An Update on Epidemiological Perspective of the Ambivalent Effects of Ultraviolet Radiation Exposure,” Front. Oncol., vol. 12, p. 866733, Mar. 2022, https://doi.org/10.3389/fonc.2022.866733.
P. W. Barnes et al., “Environmental effects of stratospheric ozone depletion, UV radiation, and interactions with climate change: UNEP Environmental Effects Assessment Panel, Update 2021,” Photochem. Photobiol. Sci., vol. 21, no. 3, pp. 275–301, Mar. 2022, https://doi.org/10.1007/s43630-022-00176-5.
R. E. Neale et al., “The effects of exposure to solar radiation on human health,” Photochem. Photobiol. Sci., vol. 22, no. 5, pp. 1011–1047, Mar. 2023, https://doi.org/10.1007/s43630-023-00375-8.
J. Robinson, R. Begum, and M. Maqbool, An Introduction to Non-Ionizing Radiation. BENTHAM SCIENCE PUBLISHERS, 2023. https://doi.org/10.2174/97898151368901230101.
G. P. Pfeifer, “Mechanisms of UV-induced mutations and skin cancer,” Genome Instab. Dis., vol. 1, no. 3, pp. 99–113, May 2020, https://doi.org/10.1007/s42764-020-00009-8.
L. Vanhaelewyn, D. Van Der Straeten, B. De Coninck, and F. Vandenbussche, “Ultraviolet Radiation From a Plant Perspective: The Plant-Microorganism Context,” Front. Plant Sci., vol. 11, p. 597642, Dec. 2020, https://doi.org/10.3389/fpls.2020.597642.
A. Mavrič Čermelj, A. Golob, K. Vogel-Mikuš, and M. Germ, “Silicon Mitigates Negative Impacts of Drought and UV-B Radiation in Plants,” Plants, vol. 11, no. 1, p. 91, Dec. 2021, https://doi.org/10.3390/plants11010091.
D. Loconsole and P. Santamaria, “UV Lighting in Horticulture: A Sustainable Tool for Improving Production Quality and Food Safety,” Horticulturae, vol. 7, no. 1, p. 9, Jan. 2021, https://doi.org/10.3390/horticulturae7010009.
E. R. Parker, “The influence of climate change on skin cancer incidence – A review of the evidence,” Int. J. Women’s Dermatology, vol. 7, no. 1, pp. 17–27, Jan. 2021, https://doi.org/10.1016/j.ijwd.2020.07.003.
I. Degtiar and S. Rose, “A Review of Generalizability and Transportability,” Annu. Rev. Stat. Its Appl., vol. 10, no. 1, pp. 501–524, Mar. 2023, https://doi.org/10.1146/annurev-statistics-042522-103837.
H. M. Levitt, “Qualitative generalization, not to the population but to the phenomenon: Reconceptualizing variation in qualitative research.,” Qual. Psychol., vol. 8, no. 1, pp. 95–110, Feb. 2021, https://doi.org/10.1037/qup0000184.
Y. Song, J. Wang, Y. Ge, and C. Xu, “An optimal parameters-based geographical detector model enhances geographic characteristics of explanatory variables for spatial heterogeneity analysis: cases with different types of spatial data,” GIScience Remote Sens., vol. 57, no. 5, pp. 593–610, Jul. 2020, https://doi.org/10.1080/15481603.2020.1760434.
H. Sulistiani and A. A. Aldino, “Decision Tree C4. 5 Algorithm For Tuition Aid Grant Program Classification (Case Study: Department Of Information System, Universitas Teknokrat Indonesia),” Edutic - Sci. J. Informatics Educ., vol. 7, no. 1, pp. 40–50, Nov. 2020, https://doi.org/10.21107/edutic.v7i1.8849.
M.-J. Jun, “A comparison of a gradient boosting decision tree, random forests, and artificial neural networks to model urban land use changes: the case of the Seoul metropolitan area,” Int. J. Geogr. Inf. Sci., vol. 35, no. 11, pp. 2149–2167, Nov. 2021, https://doi.org/10.1080/13658816.2021.1887490.
E. K. Sahin, “Assessing the predictive capability of ensemble tree methods for landslide susceptibility mapping using XGBoost, gradient boosting machine, and random forest,” SN Appl. Sci., vol. 2, no. 7, p. 1308, Jul. 2020, https://doi.org/10.1007/s42452-020-3060-1.
H. Liu, Z. Zhang, Z. Tian, and C. Lu, “Exploration for UV Aging Characteristics of Asphalt Binders based on Response Surface Methodology: Insights from the UV Aging Influencing Factors and Their Interactions,” Constr. Build. Mater., vol. 347, p. 128460, Sep. 2022, https://doi.org/10.1016/j.conbuildmat.2022.128460.
C.-Y. Wang et al., “Geographical traceability of Eucommia ulmoides leaves using attenuated total reflection Fourier transform infrared and ultraviolet-visible spectroscopy combined with chemometrics and data fusion,” Ind. Crops Prod., vol. 160, p. 113090, Feb. 2021, https://doi.org/10.1016/j.indcrop.2020.113090.
N. Yamano et al., “Long‐term Effects of 222‐nm ultraviolet radiation C Sterilizing Lamps on Mice Susceptible to Ultraviolet Radiation,” Photochem. Photobiol., vol. 96, no. 4, pp. 853–862, Jul. 2020, https://doi.org/10.1111/php.13269.
M. M. Delorme et al., “Ultraviolet radiation: An interesting technology to preserve quality and safety of milk and dairy foods,” Trends Food Sci. Technol., vol. 102, pp. 146–154, Aug. 2020, https://doi.org/10.1016/j.tifs.2020.06.001.
G. Salvadori, F. Leccese, D. Lista, C. Burattini, and F. Bisegna, “Use of smartphone apps to monitor human exposure to solar radiation: Comparison between predicted and measured UV index values,” Environ. Res., vol. 183, p. 109274, Apr. 2020, https://doi.org/10.1016/j.envres.2020.109274.
E. O. Elemo et al., “Ultraviolet Radiation Index over Abuja, Nigeria,” OALib, vol. 08, no. 09, pp. 1–17, 2021, https://doi.org/10.4236/oalib.1107924.
A. M. Chacko, F. Lagacé, and F. Jafarian, “Ultraviolet index and sun safety: are we all on the same page?,” Br. J. Dermatol., vol. 184, no. 6, pp. 1175–1176, Jun. 2021, https://doi.org/10.1111/bjd.19620.
R. Vitt et al., “UV-Index Climatology for Europe Based on Satellite Data,” Atmosphere (Basel)., vol. 11, no. 7, p. 727, Jul. 2020, https://doi.org/10.3390/atmos11070727.
S. S. Prasad, R. C. Deo, N. Downs, D. Igoe, A. V. Parisi, and J. Soar, “Cloud Affected Solar UV Prediction With Three-Phase Wavelet Hybrid Convolutional Long Short-Term Memory Network Multi-Step Forecast System,” IEEE Access, vol. 10, pp. 24704–24720, 2022, https://doi.org/10.1109/ACCESS.2022.3153475.
D. P. Igoe, A. V. Parisi, and N. J. Downs, “Cloud segmentation property extraction from total sky image repositories using Python,” Instrum. Sci. Technol., vol. 47, no. 5, pp. 522–534, Sep. 2019, https://doi.org/10.1080/10739149.2019.1603996.
R. Bajgar, A. Moukova, N. Chalupnikova, and H. Kolarova, “Differences in the Effects of Broad-Band UVA and Narrow-Band UVB on Epidermal Keratinocytes,” Int. J. Environ. Res. Public Health, vol. 18, no. 23, p. 12480, Nov. 2021, https://doi.org/10.3390/ijerph182312480.
V. A. Bahamondes Lorca, M. K. McCulloch, Ó. Ávalos‐Ovando, A. O. Govorov, F. Rahman, and S. Wu, “Characterization of UVB and UVA‐340 Lamps and Determination of Their Effects on ER Stress and DNA Damage,” Photochem. Photobiol., vol. 98, no. 5, pp. 1140–1148, Sep. 2022, https://doi.org/10.1111/php.13585.
K. Hedayat, S. Ahmad Nasrollahi, A. Firooz, H. Rastegar, and M. Dadgarnejad, “Comparison of UVA Protection Factor Measurement Protocols,” Clin. Cosmet. Investig. Dermatol., vol. Volume 13, pp. 351–358, May 2020, https://doi.org/10.2147/CCID.S244898.
F. Bernerd, T. Passeron, I. Castiel, and C. Marionnet, “The Damaging Effects of Long UVA (UVA1) Rays: A Major Challenge to Preserve Skin Health and Integrity,” Int. J. Mol. Sci., vol. 23, no. 15, p. 8243, Jul. 2022, https://doi.org/10.3390/ijms23158243.
K. J. Gromkowska‐Kępka, A. Puścion‐Jakubik, R. Markiewicz‐Żukowska, and K. Socha, “The impact of ultraviolet radiation on skin photoaging — review of in vitro studies,” J. Cosmet. Dermatol., vol. 20, no. 11, pp. 3427–3431, Nov. 2021, https://doi.org/10.1111/jocd.14033.
T. M. Ansary, M. R. Hossain, K. Kamiya, M. Komine, and M. Ohtsuki, “Inflammatory Molecules Associated with Ultraviolet Radiation-Mediated Skin Aging,” Int. J. Mol. Sci., vol. 22, no. 8, p. 3974, Apr. 2021, https://doi.org/10.3390/ijms22083974.
A. W. Schmalwieser, “Possibilities to estimate the personal UV radiation exposure from ambient UV radiation measurements,” Photochem. Photobiol. Sci., vol. 19, no. 10, pp. 1249–1261, Oct. 2020, https://doi.org/10.1039/d0pp00182a.
M. M. Mendes, K. H. Hart, S. A. Lanham-New, and P. B. Botelho, “Exploring the Impact of Individual UVB Radiation Levels on Serum 25-Hydroxyvitamin D in Women Living in High Versus Low Latitudes: A Cross-Sectional Analysis from the D-SOL Study,” Nutrients, vol. 12, no. 12, p. 3805, Dec. 2020, https://doi.org/10.3390/nu12123805.
R. Jahdi and M. Arabi, “Monitoring summer solar ultraviolet (UV) radiation on the ground level over Ardabil-Sarein, NW Iran,” ISPRS Ann. Photogramm. Remote Sens. Spat. Inf. Sci., vol. X-4/W1-202, pp. 327–333, Jan. 2023, https://doi.org/10.5194/isprs-annals-X-4-W1-2022-327-2023.
J. Turner, D. Igoe, A. V. Parisi, A. J. McGonigle, A. Amar, and L. Wainwright, “A review on the ability of smartphones to detect ultraviolet (UV) radiation and their potential to be used in UV research and for public education purposes,” Sci. Total Environ., vol. 706, p. 135873, Mar. 2020, https://doi.org/10.1016/j.scitotenv.2019.135873.
J. Bilbao and A. de Migue, “Erythemal Solar Irradiance, UVER, and UV Index from Ground-Based Data in Central Spain,” Appl. Sci., vol. 10, no. 18, p. 6589, Sep. 2020, https://doi.org/10.3390/app10186589.
J. P. Kinney, C. S. Long, and A. C. Geller, “The Ultraviolet Index: A Useful Tool.,” Dermatol. Online J., vol. 6, no. 1, Sep. 2000, https://doi.org/10.5070/D35925W4HQ.
N. Hatsusaka et al., “UV Index Does Not Predict Ocular Ultraviolet Exposure,” Transl. Vis. Sci. Technol., vol. 10, no. 7, p. 1, Jun. 2021, https://doi.org/10.1167/tvst.10.7.1.
D. R. Roshan, M. Koc, A. Abdallah, L. Martin-Pomares, R. Isaifan, and C. Fountoukis, “UV Index Forecasting under the Influence of Desert Dust: Evaluation against Surface and Satellite-Retrieved Data,” Atmosphere (Basel)., vol. 11, no. 1, p. 96, Jan. 2020, https://doi.org/10.3390/atmos11010096.
L. Marabini et al., “Effects of Vitis vinifera L. leaves extract on UV radiation damage in human keratinocytes (HaCaT),” J. Photochem. Photobiol. B Biol., vol. 204, p. 111810, Mar. 2020, https://doi.org/10.1016/j.jphotobiol.2020.111810.
E. Kanasuo, H. Siiskonen, S. Haimakainen, J. Komulainen, and I. T. Harvima, “Regular use of vitamin D supplement is associated with fewer melanoma cases compared to non-use: a cross-sectional study in 498 adult subjects at risk of skin cancers,” Melanoma Res., vol. 33, no. 2, pp. 126–135, Apr. 2023, https://doi.org/10.1097/CMR.0000000000000870.
E. M. K. Reddy, A. Gurrala, V. B. Hasitha, and K. V. R. Kumar, “Introduction to Naive Bayes and a Review on Its Subtypes with Applications,” in Bayesian Reasoning and Gaussian Processes for Machine Learning Applications, Boca Raton: Chapman and Hall/CRC, 2022, pp. 1–14. https://doi.org/10.1201/9781003164265-1.
L. M. Sinaga, Sawaluddin, and S. Suwilo, “Analysis of classification and Naïve Bayes algorithm k-nearest neighbor in data mining,” IOP Conf. Ser. Mater. Sci. Eng., vol. 725, no. 1, p. 012106, Jan. 2020, https://doi.org/10.1088/1757-899X/725/1/012106.
S. S. Bafjaish, “Comparative analysis of Naive Bayesian techniques in health-related for classification task,” J. Soft Comput. Data Min., vol. 1, no. 2, pp. 1–10, 2020, [Online]. Available: https://publisher.uthm.edu.my/ojs/index.php/jscdm/article/view/7144
V. Jackins, S. Vimal, M. Kaliappan, and M. Y. Lee, “AI-based smart prediction of clinical disease using random forest classifier and Naive Bayes,” J. Supercomput., vol. 77, no. 5, pp. 5198–5219, May 2021, https://doi.org/10.1007/s11227-020-03481-x.
J. Galopo Perez and E. S. Perez, “Predicting Student Program Completion Using Naïve Bayes Classification Algorithm,” Int. J. Mod. Educ. Comput. Sci., vol. 13, no. 3, pp. 57–67, Jun. 2021, https://doi.org/10.5815/ijmecs.2021.03.05.
M. M. Taye, “Understanding of Machine Learning with Deep Learning: Architectures, Workflow, Applications and Future Directions,” Computers, vol. 12, no. 5, p. 91, Apr. 2023, https://doi.org/10.3390/computers12050091.
R. Pugliese, S. Regondi, and R. Marini, “Machine learning-based approach: global trends, research directions, and regulatory standpoints,” Data Sci. Manag., vol. 4, pp. 19–29, Dec. 2021, https://doi.org/10.1016/j.dsm.2021.12.002.
A. Ali, W. Samara, D. Alhaddad, A. Ware, and O. A. Saraereh, “Human Activity and Motion Pattern Recognition within Indoor Environment Using Convolutional Neural Networks Clustering and Naive Bayes Classification Algorithms,” Sensors, vol. 22, no. 3, p. 1016, Jan. 2022, https://doi.org/10.3390/s22031016.
D. Iskandaryan, F. Ramos, and S. Trilles, “Air Quality Prediction in Smart Cities Using Machine Learning Technologies Based on Sensor Data: A Review,” Appl. Sci., vol. 10, no. 7, p. 2401, Apr. 2020, https://doi.org/10.3390/app10072401.
Y. Sun and Y. Ma, “Application of Classification Algorithm Based on Naive Bayes in Data Analysis of Fitness Test,” J. Phys. Conf. Ser., vol. 1648, no. 4, p. 042078, Oct. 2020, https://doi.org/10.1088/1742-6596/1648/4/042078.
X. Liu, D. Lu, A. Zhang, Q. Liu, and G. Jiang, “Data-Driven Machine Learning in Environmental Pollution: Gains and Problems,” Environ. Sci. Technol., vol. 56, no. 4, pp. 2124–2133, Feb. 2022, https://doi.org/10.1021/acs.est.1c06157.
L. Cui and Z. Liu, “Synergy between research on ensemble perception, data visualization, and statistics education: A tutorial review,” Attention, Perception, Psychophys., vol. 83, no. 3, pp. 1290–1311, Apr. 2021, https://doi.org/10.3758/s13414-020-02212-x.
H. Xu, A. Berres, Y. Liu, M. R. Allen-Dumas, and J. Sanyal, “An overview of visualization and visual analytics applications in water resources management,” Environ. Model. Softw., vol. 153, p. 105396, Jul. 2022, https://doi.org/10.1016/j.envsoft.2022.105396.
B. Charbuty and A. Abdulazeez, “Classification Based on Decision Tree Algorithm for Machine Learning,” J. Appl. Sci. Technol. Trends, vol. 2, no. 01, pp. 20–28, Mar. 2021, https://doi.org/10.38094/jastt20165.
H. Lu and X. Ma, “Hybrid decision tree-based machine learning models for short-term water quality prediction,” Chemosphere, vol. 249, p. 126169, Jun. 2020, https://doi.org/10.1016/j.chemosphere.2020.126169.
T. Thomas, A. P. Vijayaraghavan, and S. Emmanuel, Machine Learning Approaches in Cyber Security Analytics. Singapore: Springer Singapore, 2020. https://doi.org/10.1007/978-981-15-1706-8.
S. Mishra, P. K. Mallick, H. K. Tripathy, A. K. Bhoi, and A. González-Briones, “Performance Evaluation of a Proposed Machine Learning Model for Chronic Disease Datasets Using an Integrated Attribute Evaluator and an Improved Decision Tree Classifier,” Appl. Sci., vol. 10, no. 22, p. 8137, Nov. 2020, https://doi.org/10.3390/app10228137.
A. N. Elmachtoub, J. C. N. Liang, and R. McNellis, “Decision trees for decision-making under the predict-then-optimize framework,” in International conference on machine learning, PMLR, 2020, pp. 2858–2867. [Online]. Available: https://proceedings.mlr.press/v119/elmachtoub20a.html
A. D. Woods et al., Missing Data and Multiple Imputation Decision Tree. 2021. https://doi.org/10.31234/osf.io/mdw5r.
T. Emmanuel, T. Maupong, D. Mpoeleng, T. Semong, B. Mphago, and O. Tabona, “A survey on missing data in machine learning,” J. Big Data, vol. 8, no. 1, p. 140, Oct. 2021, https://doi.org/10.1186/s40537-021-00516-9.
O. Sagi and L. Rokach, “Explainable decision forest: Transforming a decision forest into an interpretable tree,” Inf. Fusion, vol. 61, pp. 124–138, Sep. 2020, https://doi.org/10.1016/j.inffus.2020.03.013.
G. R. Yang and X.-J. Wang, “Artificial Neural Networks for Neuroscientists: A Primer,” Neuron, vol. 107, no. 6, pp. 1048–1070, Sep. 2020, https://doi.org/10.1016/j.neuron.2020.09.005.
A. Mehonic, A. Sebastian, B. Rajendran, O. Simeone, E. Vasilaki, and A. J. Kenyon, “Memristors—From In‐Memory Computing, Deep Learning Acceleration, and Spiking Neural Networks to the Future of Neuromorphic and Bio‐Inspired Computing,” Adv. Intell. Syst., vol. 2, no. 11, p. 2000085, Nov. 2020, https://doi.org/10.1002/aisy.202000085.
A. Thakur, “Fundamentals of Neural Networks,” Int. J. Res. Appl. Sci. Eng. Technol., vol. 9, no. VIII, pp. 407–426, Aug. 2021, https://doi.org/10.22214/ijraset.2021.37362.
M. Rithani, R. P. Kumar, and S. Doss, “A review on big data based on deep neural network approaches,” Artif. Intell. Rev., vol. 56, no. 12, pp. 14765–14801, Dec. 2023, https://doi.org/10.1007/s10462-023-10512-5.
T. P. Lillicrap, A. Santoro, L. Marris, C. J. Akerman, and G. Hinton, “Backpropagation and the brain,” Nat. Rev. Neurosci., vol. 21, no. 6, pp. 335–346, Jun. 2020, https://doi.org/10.1038/s41583-020-0277-3.
R. Dastres and M. Soori, “Artificial neural network systems,” Int. J. Imaging Robot., vol. 21, no. 2, pp. 13–25, 2021, [Online]. Available: https://hal.science/hal-03349542
O. A. Montesinos López, A. Montesinos López, and J. Crossa, “Fundamentals of Artificial Neural Networks and Deep Learning,” in Multivariate Statistical Machine Learning Methods for Genomic Prediction, Cham: Springer International Publishing, 2022, pp. 379–425. https://doi.org/10.1007/978-3-030-89010-0_10.
W. Samek, G. Montavon, S. Lapuschkin, C. J. Anders, and K.-R. Muller, “Explaining Deep Neural Networks and Beyond: A Review of Methods and Applications,” Proc. IEEE, vol. 109, no. 3, pp. 247–278, Mar. 2021, https://doi.org/10.1109/JPROC.2021.3060483.
D. A. Pisner and D. M. Schnyer, “Support vector machine,” in Machine Learning, Elsevier, 2020, pp. 101–121. https://doi.org/10.1016/B978-0-12-815739-8.00006-7.
J. Cervantes, F. Garcia-Lamont, L. Rodríguez-Mazahua, and A. Lopez, “A comprehensive survey on support vector machine classification: Applications, challenges and trends,” Neurocomputing, vol. 408, pp. 189–215, Sep. 2020, https://doi.org/10.1016/j.neucom.2019.10.118.
A. Rizwan, N. Iqbal, R. Ahmad, and D.-H. Kim, “WR-SVM Model Based on the Margin Radius Approach for Solving the Minimum Enclosing Ball Problem in Support Vector Machine Classification,” Appl. Sci., vol. 11, no. 10, p. 4657, May 2021, https://doi.org/10.3390/app11104657.
D. Hsu, V. Muthukumar, and J. Xu, “On the proliferation of support vectors in high dimensions,” in International Conference on Artificial Intelligence and Statistics, PMLR, 2021, pp. 91–99. [Online]. Available: https://proceedings.mlr.press/v130/hsu21a.html
N. Ardeshir, C. Sanford, and D. J. Hsu, “Support vector machines and linear regression coincide with very high-dimensional features,” Adv. Neural Inf. Process. Syst., vol. 34, pp. 4907–4918, 2021, [Online]. Available: https://proceedings.neurips.cc/paper/2021/file/26d4b4313a7e5828856bc0791fca39a2-Paper.pdf
P. El Kafrawy, H. Fathi, M. Qaraad, A. K. Kelany, and X. Chen, “An Efficient SVM-Based Feature Selection Model for Cancer Classification Using High-Dimensional Microarray Data,” IEEE Access, vol. 9, pp. 155353–155369, 2021, https://doi.org/10.1109/ACCESS.2021.3123090.
E. Y. Boateng, J. Otoo, and D. A. Abaye, “Basic Tenets of Classification Algorithms K-Nearest-Neighbor, Support Vector Machine, Random Forest and Neural Network: A Review,” J. Data Anal. Inf. Process., vol. 08, no. 04, pp. 341–357, 2020, https://doi.org/10.4236/jdaip.2020.84020.
M. Hou and C. Kambhampati, “Locally fitting hyperplanes to high-dimensional data,” Neural Comput. Appl., vol. 34, no. 11, pp. 8885–8896, Jun. 2022, https://doi.org/10.1007/s00521-022-06909-y.
K. Wang and C. Thrampoulidis, “Binary Classification of Gaussian Mixtures: Abundance of Support Vectors, Benign Overfitting, and Regularization,” SIAM J. Math. Data Sci., vol. 4, no. 1, pp. 260–284, Mar. 2022, https://doi.org/10.1137/21M1415121.
V. Vapnik and R. Izmailov, “Reinforced SVM method and memorization mechanisms,” Pattern Recognit., vol. 119, p. 108018, Nov. 2021, https://doi.org/10.1016/j.patcog.2021.108018.
F. Nie, W. Zhu, and X. Li, “Decision Tree SVM: An extension of linear SVM for non-linear classification,” Neurocomputing, vol. 401, pp. 153–159, Aug. 2020, https://doi.org/10.1016/j.neucom.2019.10.051.
S. Shojae Chaeikar, A. A. Manaf, A. A. Alarood, and M. Zamani, “PFW: Polygonal Fuzzy Weighted—An SVM Kernel for the Classification of Overlapping Data Groups,” Electronics, vol. 9, no. 4, p. 615, Apr. 2020, https://doi.org/10.3390/electronics9040615.
F. Farshadmoghadam, H. D. Azodi, and M. R. Yaghouti, “An Efficient Alternative Kernel of Gaussian Radial Basis Function for Solving Nonlinear Integro-Differential Equations,” Iran. J. Sci. Technol. Trans. A Sci., vol. 46, no. 3, pp. 869–881, Jun. 2022, https://doi.org/10.1007/s40995-022-01286-6.
K. Thurnhofer-Hemsi, E. López-Rubio, M. A. Molina-Cabello, and K. Najarian, “Radial basis function kernel optimization for Support Vector Machine classifiers,” Jul. 2020, [Online]. Available: http://arxiv.org/abs/2007.08233
I. S. Al-Mejibli, J. K. Alwan, and D. H. Abd, “The effect of gamma value on support vector machine performance with different kernels,” Int. J. Electr. Comput. Eng., vol. 10, no. 5, p. 5497, Oct. 2020, https://doi.org/10.11591/ijece.v10i5.pp5497-5506.
Q. Gu, Y. Chang, X. Li, Z. Chang, and Z. Feng, “A novel F-SVM based on FOA for improving SVM performance,” Expert Syst. Appl., vol. 165, p. 113713, Mar. 2021, https://doi.org/10.1016/j.eswa.2020.113713.
R.-C. Chen, C. Dewi, S.-W. Huang, and R. E. Caraka, “Selecting critical features for data classification based on machine learning methods,” J. Big Data, vol. 7, no. 1, p. 52, Dec. 2020, https://doi.org/10.1186/s40537-020-00327-4.
N. Rtayli and N. Enneya, “Enhanced credit card fraud detection based on SVM-recursive feature elimination and hyper-parameters optimization,” J. Inf. Secur. Appl., vol. 55, p. 102596, Dec. 2020, https://doi.org/10.1016/j.jisa.2020.102596.
T. Wang, L. Zhang, and W. Hu, “Bridging deep and multiple kernel learning: A review,” Inf. Fusion, vol. 67, pp. 3–13, Mar. 2021, https://doi.org/10.1016/j.inffus.2020.10.002.
M. A. Deif, A. A. A. Solyman, M. H. Alsharif, S. Jung, and E. Hwang, “A Hybrid Multi-Objective Optimizer-Based SVM Model for Enhancing Numerical Weather Prediction: A Study for the Seoul Metropolitan Area,” Sustainability, vol. 14, no. 1, p. 296, Dec. 2021, https://doi.org/10.3390/su14010296.
B. Azari, K. Hassan, J. Pierce, and S. Ebrahimi, “Evaluation of Machine Learning Methods Application in Temperature Prediction,” Comput. Res. Prog. Appl. Sci. Eng., vol. 8, no. 1, pp. 1–12, 2022, https://doi.org/10.52547/crpase.8.1.2747.
N. Sultana, “Predicting sun protection measures against skin diseases using machine learning approaches,” J. Cosmet. Dermatol., vol. 21, no. 2, pp. 758–769, Feb. 2022, https://doi.org/10.1111/jocd.14120.
M. Jafari Gukeh, S. Moitra, A. N. Ibrahim, S. Derrible, and C. M. Megaridis, “Machine Learning Prediction of TiO 2 -Coating Wettability Tuned via UV Exposure,” ACS Appl. Mater. Interfaces, vol. 13, no. 38, pp. 46171–46179, Sep. 2021, https://doi.org/10.1021/acsami.1c13262.
K. P. Sinaga and M.-S. Yang, “Unsupervised K-Means Clustering Algorithm,” IEEE Access, vol. 8, pp. 80716–80727, 2020, https://doi.org/10.1109/ACCESS.2020.2988796.
K. P. Sinaga, I. Hussain, and M.-S. Yang, “Entropy K-Means Clustering With Feature Reduction Under Unknown Number of Clusters,” IEEE Access, vol. 9, pp. 67736–67751, 2021, https://doi.org/10.1109/ACCESS.2021.3077622.
E. U. Oti, M. O. Olusola, F. C. Eze, and S. U. Enogwe, “Comprehensive Review of K-Means Clustering Algorithms,” Int. J. Adv. Sci. Res. Eng., vol. 07, no. 08, pp. 64–69, 2021, https://doi.org/10.31695/IJASRE.2021.34050.
R. Qaddoura, H. Faris, and I. Aljarah, “An efficient clustering algorithm based on the k-nearest neighbors with an indexing ratio,” Int. J. Mach. Learn. Cybern., vol. 11, no. 3, pp. 675–714, Mar. 2020, https://doi.org/10.1007/s13042-019-01027-z.
S. Daoudi, C. M. A. Zouaoui, M. C. El-Mezouar, and N. Taleb, “Parallelization of the K-Means++ Clustering Algorithm,” Ingénierie des systèmes d Inf., vol. 26, no. 1, pp. 59–66, Feb. 2021, https://doi.org/10.18280/isi.260106.
V. Romanuke, “Random centroid initialization for improving centroid-based clustering,” Decis. Mak. Appl. Manag. Eng., vol. 6, no. 2, pp. 734–746, Dec. 2023, https://doi.org/10.31181/dmame622023742.
A. K. Abdalameer, M. Alswaitti, A. A. Alsudani, and N. A. M. Isa, “A new validity clustering index-based on finding new centroid positions using the mean of clustered data to determine the optimum number of clusters,” Expert Syst. Appl., vol. 191, p. 116329, Apr. 2022, https://doi.org/10.1016/j.eswa.2021.116329.
Y. Li, X. Zhou, J. Gu, K. Guo, and W. Deng, “A Novel K-Means Clustering Method for Locating Urban Hotspots Based on Hybrid Heuristic Initialization,” Appl. Sci., vol. 12, no. 16, p. 8047, Aug. 2022, https://doi.org/10.3390/app12168047.
A. F. R. Guarda, N. M. M. Rodrigues, and F. Pereira, “Constant Size Point Cloud Clustering: A Compact, Non-Overlapping Solution,” IEEE Trans. Multimed., vol. 23, pp. 77–91, 2021, https://doi.org/10.1109/TMM.2020.2974325.
A. M. Ikotun, A. E. Ezugwu, L. Abualigah, B. Abuhaija, and J. Heming, “K-means clustering algorithms: A comprehensive review, variants analysis, and advances in the era of big data,” Inf. Sci. (Ny)., vol. 622, pp. 178–210, Apr. 2023, https://doi.org/10.1016/j.ins.2022.11.139.
P. Govender and V. Sivakumar, “Application of k-means and hierarchical clustering techniques for analysis of air pollution: A review (1980–2019),” Atmos. Pollut. Res., vol. 11, no. 1, pp. 40–56, Jan. 2020, https://doi.org/10.1016/j.apr.2019.09.009.
W. Zeng, Y. Jiang, Z. Huo, and K. Hu, “Clustering Analysis of Extreme Temperature Based on K-means Algorithm,” 2020, pp. 523–533. https://doi.org/10.1007/978-3-030-57881-7_46.
P. Stoker, G. Tian, and J. Y. Kim, “Analysis of Variance (ANOVA),” in Basic Quantitative Research Methods for Urban Planners, Routledge, 2020, pp. 197–219. https://doi.org/10.4324/9780429325021-11.
K. G. Burkart et al., “Estimating the cause-specific relative risks of non-optimal temperature on daily mortality: a two-part modelling approach applied to the Global Burden of Disease Study,” Lancet, vol. 398, no. 10301, pp. 685–697, Aug. 2021, https://doi.org/10.1016/S0140-6736(21)01700-1.
G. Arnqvist, “Mixed Models Offer No Freedom from Degrees of Freedom,” Trends Ecol. Evol., vol. 35, no. 4, pp. 329–335, Apr. 2020, https://doi.org/10.1016/j.tree.2019.12.004.
D. Shi, C. DiStefano, A. Maydeu-Olivares, and T. Lee, “Evaluating SEM Model Fit with Small Degrees of Freedom,” Multivariate Behav. Res., vol. 57, no. 2–3, pp. 179–207, May 2022, https://doi.org/10.1080/00273171.2020.1868965.
G. Pavlov, A. Maydeu-Olivares, and D. Shi, “Using the Standardized Root Mean Squared Residual (SRMR) to Assess Exact Fit in Structural Equation Models,” Educ. Psychol. Meas., vol. 81, no. 1, pp. 110–130, Feb. 2021, https://doi.org/10.1177/0013164420926231.
M. A. Mansournia, M. Nazemipour, A. I. Naimi, G. S. Collins, and M. J. Campbell, “Reflection on modern methods: demystifying robust standard errors for epidemiologists,” Int. J. Epidemiol., vol. 50, no. 1, pp. 346–351, Mar. 2021, https://doi.org/10.1093/ije/dyaa260.
D. S. K. Karunasingha, “Root mean square error or mean absolute error? Use their ratio as well,” Inf. Sci. (Ny)., vol. 585, pp. 609–629, Mar. 2022, https://doi.org/10.1016/j.ins.2021.11.036.
T. Kunz and T. Laepple, “Frequency-Dependent Estimation of Effective Spatial Degrees of Freedom,” J. Clim., vol. 34, no. 18, pp. 7373–7388, Sep. 2021, https://doi.org/10.1175/JCLI-D-20-0228.1.
L. Tognetti et al., “UVA‐1 phototherapy as adjuvant treatment for eosinophilic fasciitis: in vitro and in vivo functional characterization,” Int. J. Dermatol., vol. 61, no. 6, pp. 718–726, Jun. 2022, https://doi.org/10.1111/ijd.16003.
B. O. Saguie, R. L. Martins, A. de S. da Fonseca, B. Romana-Souza, and A. Monte-Alto-Costa, “An ex vivo model of human skin photoaging induced by UVA radiation compatible with summer exposure in Brazil,” J. Photochem. Photobiol. B Biol., vol. 221, p. 112255, Aug. 2021, https://doi.org/10.1016/j.jphotobiol.2021.112255.
N. Ibrahim and A. B. Abdullahi, “Analysis of Variance (ANOVA) Randomized Block Design (RBD) to Test the Variability of Three Different Types of Fertilizers (NPK, UREA and SSP) on Millet Production,” African J. Agric. Sci. Food Res., vol. 9, no. 1, pp. 1–10, 2023, [Online]. Available: https://publications.afropolitanjournals.com/index.php/ajasfr/article/view/326
A. A. T., “Analysis of Variance: The Fundamental Concepts and Application with R,” Int. J. Math. Comput. Res., vol. 09, no. 10, pp. 2408–2422, Oct. 2021, https://doi.org/10.47191/ijmcr/v9i10.04.
S. Farhana, “Classification of Academic Performance for University Research Evaluation by Implementing Modified Naive Bayes Algorithm,” Procedia Comput. Sci., vol. 194, pp. 224–228, 2021, https://doi.org/10.1016/j.procs.2021.10.077.
K. Maswadi, N. A. Ghani, S. Hamid, and M. B. Rasheed, “Human activity classification using Decision Tree and Naïve Bayes classifiers,” Multimed. Tools Appl., vol. 80, no. 14, pp. 21709–21726, Jun. 2021, https://doi.org/10.1007/s11042-020-10447-x.
Y. Ying, T. N. Mursitama, Shidarta, and Lohansen, “Effectiveness of the News Text Classification Test Using the Naïve Bayes’ Classification Text Mining Method,” J. Phys. Conf. Ser., vol. 1764, no. 1, p. 012105, Feb. 2021, https://doi.org/10.1088/1742-6596/1764/1/012105.
N. Wijaya, “Evaluation of Naïve Bayes and Chi-Square performance for Classification of Occupancy House,” Int. J. Informatics Comput., vol. 1, no. 2, p. 46, Feb. 2020, https://doi.org/10.35842/ijicom.v1i2.20.
S. Jayachitra and A. Prasanth, “Multi-Feature Analysis for Automated Brain Stroke Classification Using Weighted Gaussian Naïve Bayes Classifier,” J. Circuits, Syst. Comput., vol. 30, no. 10, p. 2150178, Aug. 2021, https://doi.org/10.1142/S0218126621501784.
T. N. Viet, H. Le Minh, L. C. Hieu, and T. H. Anh, “The Naïve Bayes algorithm for learning data analytics,” Indian J. Comput. Sci. Eng., vol. 12, no. 4, pp. 1038–1043, Aug. 2021, https://doi.org/10.21817/indjcse/2021/v12i4/211204191.
S. Tangirala, “Evaluating the Impact of GINI Index and Information Gain on Classification using Decision Tree Classifier Algorithm*,” Int. J. Adv. Comput. Sci. Appl., vol. 11, no. 2, pp. 612–619, 2020, https://doi.org/10.14569/IJACSA.2020.0110277.
M. Bansal, A. Goyal, and A. Choudhary, “A comparative analysis of K-Nearest Neighbor, Genetic, Support Vector Machine, Decision Tree, and Long Short Term Memory algorithms in machine learning,” Decis. Anal. J., vol. 3, p. 100071, Jun. 2022, https://doi.org/10.1016/j.dajour.2022.100071.
T. O. Hodson, T. M. Over, and S. S. Foks, “Mean Squared Error, Deconstructed,” J. Adv. Model. Earth Syst., vol. 13, no. 12, p. e2021MS002681, Dec. 2021, https://doi.org/10.1029/2021MS002681.
X. Zhu and S. Tang, “A Branch-and-Bound Algorithm for Building Optimal Data Gathering Tree in Wireless Sensor Networks,” INFORMS J. Comput., vol. 33, no. 4, pp. 1446–1460, Feb. 2021, https://doi.org/10.1287/ijoc.2020.1012.
G. Zarpellon, J. Jo, A. Lodi, and Y. Bengio, “Parameterizing Branch-and-Bound Search Trees to Learn Branching Policies,” Proc. AAAI Conf. Artif. Intell., vol. 35, no. 5, pp. 3931–3939, May 2021, https://doi.org/10.1609/aaai.v35i5.16512.
G. James, D. Witten, T. Hastie, R. Tibshirani, and J. Taylor, “Tree-Based Methods,” 2023, pp. 331–366. https://doi.org/10.1007/978-3-031-38747-0_8.
D. Kumar and N. A. Priyanka, “Decision tree classifier: a detailed survey,” Int. J. Inf. Decis. Sci., vol. 12, no. 3, p. 246, 2020, https://doi.org/10.1504/IJIDS.2020.10029122.
M. M. Ghiasi, S. Zendehboudi, and A. A. Mohsenipour, “Decision tree-based diagnosis of coronary artery disease: CART model,” Comput. Methods Programs Biomed., vol. 192, p. 105400, Aug. 2020, https://doi.org/10.1016/j.cmpb.2020.105400.
A. Singh, V. Kotiyal, S. Sharma, J. Nagar, and C.-C. Lee, “A Machine Learning Approach to Predict the Average Localization Error With Applications to Wireless Sensor Networks,” IEEE Access, vol. 8, pp. 208253–208263, 2020, https://doi.org/10.1109/ACCESS.2020.3038645.
I. Ahmad, M. U. Akhtar, S. Noor, and A. Shahnaz, “Missing Link Prediction using Common Neighbor and Centrality based Parameterized Algorithm,” Sci. Rep., vol. 10, no. 1, p. 364, Jan. 2020, https://doi.org/10.1038/s41598-019-57304-y.
B. Albanna, R. Heeks, A. Pawelke, J. Boy, J. Handl, and A. Gluecker, “Data-powered positive deviance: Combining traditional and non-traditional data to identify and characterise development-related outperformers,” Dev. Eng., vol. 7, p. 100090, 2022, https://doi.org/10.1016/j.deveng.2021.100090.
A. Shehadeh, O. Alshboul, R. E. Al Mamlook, and O. Hamedat, “Machine learning models for predicting the residual value of heavy construction equipment: An evaluation of modified decision tree, LightGBM, and XGBoost regression,” Autom. Constr., vol. 129, p. 103827, Sep. 2021, https://doi.org/10.1016/j.autcon.2021.103827.
Y. Wang et al., “Visualizing Element Migration over Bifunctional Metal‐Zeolite Catalysts and its Impact on Catalysis,” Angew. Chemie Int. Ed., vol. 60, no. 32, pp. 17735–17743, Aug. 2021, https://doi.org/10.1002/anie.202107264.
Y. Chen, L. Song, Y. Liu, L. Yang, and D. Li, “A Review of the Artificial Neural Network Models for Water Quality Prediction,” Appl. Sci., vol. 10, no. 17, p. 5776, Aug. 2020, https://doi.org/10.3390/app10175776.
Q. Liu, M. F. Iqbal, J. Yang, X. Lu, P. Zhang, and M. Rauf, “Prediction of chloride diffusivity in concrete using artificial neural network: Modelling and performance evaluation,” Constr. Build. Mater., vol. 268, p. 121082, Jan. 2021, https://doi.org/10.1016/j.conbuildmat.2020.121082.
J. Jawad, A. H. Hawari, and S. Javaid Zaidi, “Artificial neural network modeling of wastewater treatment and desalination using membrane processes: A review,” Chem. Eng. J., vol. 419, p. 129540, Sep. 2021, https://doi.org/10.1016/j.cej.2021.129540.
D.-H. Lee, Y.-T. Kim, and S.-R. Lee, “Shallow Landslide Susceptibility Models Based on Artificial Neural Networks Considering the Factor Selection Method and Various Non-Linear Activation Functions,” Remote Sens., vol. 12, no. 7, p. 1194, Apr. 2020, https://doi.org/10.3390/rs12071194.
A. A. Alkhouly, A. Mohammed, and H. A. Hefny, “Improving the Performance of Deep Neural Networks Using Two Proposed Activation Functions,” IEEE Access, vol. 9, pp. 82249–82271, 2021, https://doi.org/10.1109/ACCESS.2021.3085855.
Y. Wang, Y. Li, Y. Song, and X. Rong, “The Influence of the Activation Function in a Convolution Neural Network Model of Facial Expression Recognition,” Appl. Sci., vol. 10, no. 5, p. 1897, Mar. 2020, https://doi.org/10.3390/app10051897.
S. Namasudra, S. Dhamodharavadhani, and R. Rathipriya, “Nonlinear Neural Network Based Forecasting Model for Predicting COVID-19 Cases,” Neural Process. Lett., vol. 55, no. 1, pp. 171–191, Feb. 2023, https://doi.org/10.1007/s11063-021-10495-w.
R. Pal, A. A. Sekh, S. Kar, and D. K. Prasad, “Neural Network Based Country Wise Risk Prediction of COVID-19,” Appl. Sci., vol. 10, no. 18, p. 6448, Sep. 2020, https://doi.org/10.3390/app10186448.
J. Elliott et al., “Predictive Accuracy of a Polygenic Risk Score–Enhanced Prediction Model vs a Clinical Risk Score for Coronary Artery Disease,” JAMA, vol. 323, no. 7, p. 636, Feb. 2020, https://doi.org/10.1001/jama.2019.22241.
R. Valavi, G. Guillera‐Arroita, J. J. Lahoz‐Monfort, and J. Elith, “Predictive performance of presence‐only species distribution models: a benchmark study with reproducible code,” Ecol. Monogr., vol. 92, no. 1, p. e01486, Feb. 2022, https://doi.org/10.1002/ecm.1486.
Z. Shen, H. Yang, and S. Zhang, “Neural network approximation: Three hidden layers are enough,” Neural Networks, vol. 141, pp. 160–173, Sep. 2021, https://doi.org/10.1016/j.neunet.2021.04.011.
T. Szandała, “Review and Comparison of Commonly Used Activation Functions for Deep Neural Networks,” 2021, pp. 203–224. https://doi.org/10.1007/978-981-15-5495-7_11.
S. H. Bhojani and N. Bhatt, “Wheat crop yield prediction using new activation functions in neural network,” Neural Comput. Appl., vol. 32, no. 17, pp. 13941–13951, Sep. 2020, https://doi.org/10.1007/s00521-020-04797-8.
J. You, K. H. J. Leskovec, and S. Xie, “Graph structure of neural networks,” in International Conference on Machine Learning, PMLR, 2020, pp. 10881–10891. https://doi.org/10.48550/arXiv.2007.06559.
M. M. Taye, “Theoretical Understanding of Convolutional Neural Network: Concepts, Architectures, Applications, Future Directions,” Computation, vol. 11, no. 3, p. 52, Mar. 2023, https://doi.org/10.3390/computation11030052.
J. Senk et al., “Connectivity concepts in neuronal network modeling,” PLOS Comput. Biol., vol. 18, no. 9, p. e1010086, Sep. 2022, https://doi.org/10.1371/journal.pcbi.1010086.
K. Lenk, B. Genocchi, M. T. Barros, and J. A. K. Hyttinen, “Larger Connection Radius Increases Hub Astrocyte Number in a 3-D Neuron–Astrocyte Network Model,” IEEE Trans. Mol. Biol. Multi-Scale Commun., vol. 7, no. 2, pp. 83–88, Jun. 2021, https://doi.org/10.1109/TMBMC.2021.3054890.
L. Luo, “Architectures of neuronal circuits,” Science (80-. )., vol. 373, no. 6559, p. eabg7285, Sep. 2021, https://doi.org/10.1126/science.abg7285.
S. Chung and L. F. Abbott, “Neural population geometry: An approach for understanding biological and artificial neural networks,” Curr. Opin. Neurobiol., vol. 70, pp. 137–144, Oct. 2021, https://doi.org/10.1016/j.conb.2021.10.010.
L. Das, A. Sivaram, and V. Venkatasubramanian, “Hidden representations in deep neural networks: Part 2. Regression problems,” Comput. Chem. Eng., vol. 139, p. 106895, Aug. 2020, https://doi.org/10.1016/j.compchemeng.2020.106895.
M. Uzair and N. Jamil, “Effects of Hidden Layers on the Efficiency of Neural networks,” in 2020 IEEE 23rd International Multitopic Conference (INMIC), Nov. 2020, pp. 1–6. https://doi.org/10.1109/INMIC50486.2020.9318195.
H. M. D. Kabir et al., “SpinalNet: Deep Neural Network With Gradual Input,” IEEE Trans. Artif. Intell., vol. 4, no. 5, pp. 1165–1177, Oct. 2023, https://doi.org/10.1109/TAI.2022.3185179.
M. G. M. Abdolrasol et al., “Artificial Neural Networks Based Optimization Techniques: A Review,” Electronics, vol. 10, no. 21, p. 2689, Nov. 2021, https://doi.org/10.3390/electronics10212689.
A. A. Movassagh et al., “Artificial neural networks training algorithm integrating invasive weed optimization with differential evolutionary model,” J. Ambient Intell. Humaniz. Comput., vol. 14, no. 5, pp. 6017–6025, May 2023, https://doi.org/10.1007/s12652-020-02623-6.
S. Kiliçarslan and M. Celik, “RSigELU: A nonlinear activation function for deep neural networks,” Expert Syst. Appl., vol. 174, p. 114805, Jul. 2021, https://doi.org/10.1016/j.eswa.2021.114805.
N. Kulathunga, N. R. Ranasinghe, D. Vrinceanu, Z. Kinsman, L. Huang, and Y. Wang, “Effects of the Nonlinearity in Activation Functions on the Performance of Deep Learning Models,” Oct. 2020, https://doi.org/10.48550/arXiv.2010.07359.
F. Emmert-Streib, Z. Yang, H. Feng, S. Tripathi, and M. Dehmer, “An Introductory Review of Deep Learning for Prediction Models With Big Data,” Front. Artif. Intell., vol. 3, p. 4, Feb. 2020, https://doi.org/10.3389/frai.2020.00004.
M. N. Fekri, H. Patel, K. Grolinger, and V. Sharma, “Deep learning for load forecasting with smart meter data: Online Adaptive Recurrent Neural Network,” Appl. Energy, vol. 282, p. 116177, Jan. 2021, https://doi.org/10.1016/j.apenergy.2020.116177.
J. L. Zambrano, J. A. L. Torralbo, and C. R. Morales, “Early prediction of student learning performance through data mining: A systematic review,” Psicothema, 2021, https://doi.org/10.7334/psicothema2021.62.
H. Meyer and E. Pebesma, “Predicting into unknown space? Estimating the area of applicability of spatial prediction models,” Methods Ecol. Evol., vol. 12, no. 9, pp. 1620–1633, Sep. 2021, https://doi.org/10.1111/2041-210X.13650.
J. Marulanda-Durango, A. Escobar-Mejía, A. Alzate-Gómez, and M. Álvarez-López, “A Support Vector machine-Based method for parameter estimation of an electric arc furnace model,” Electr. Power Syst. Res., vol. 196, p. 107228, Jul. 2021, https://doi.org/10.1016/j.epsr.2021.107228.
G. Kavithaa and S. Bhuvaneswari, “Kernel Linkage Support Vector Regression For Stock Market Index Prediction And Analysis,” Turkish J. Comput. Math. Educ., vol. 12, no. 12, pp. 3289–3300, 2021, https://doi.org/10.17762/turcomat.v12i12.8008.
N. Karunanithi, D. Whitley, and Y. K. Malaiya, “Using neural networks in reliability prediction,” IEEE Softw., vol. 9, no. 4, pp. 53–59, Jul. 1992, https://doi.org/10.1109/52.143107.
Y. Koçak and G. Üstündağ Şiray, “New activation functions for single layer feedforward neural network,” Expert Syst. Appl., vol. 164, p. 113977, Feb. 2021, https://doi.org/10.1016/j.eswa.2020.113977.
M. A. Chandra and S. S. Bedi, “Survey on SVM and their application in image classification,” Int. J. Inf. Technol., vol. 13, no. 5, pp. 1–11, Oct. 2021, https://doi.org/10.1007/s41870-017-0080-1.
J. Shao, X. Liu, and W. He, “Kernel Based Data-Adaptive Support Vector Machines for Multi-Class Classification,” Mathematics, vol. 9, no. 9, p. 936, Apr. 2021, https://doi.org/10.3390/math9090936.
L. Mohan, J. Pant, P. Suyal, and A. Kumar, “Support Vector Machine Accuracy Improvement with Classification,” in 2020 12th International Conference on Computational Intelligence and Communication Networks (CICN), Sep. 2020, pp. 477–481. https://doi.org/10.1109/CICN49253.2020.9242572.
R. Guido, M. C. Groccia, and D. Conforti, “A hyper-parameter tuning approach for cost-sensitive support vector machine classifiers,” Soft Comput., vol. 27, no. 18, pp. 12863–12881, Sep. 2023, https://doi.org/10.1007/s00500-022-06768-8.
N. Tran, J.-G. Schneider, I. Weber, and A. K. Qin, “Hyper-parameter optimization in classification: To-do or not-to-do,” Pattern Recognit., vol. 103, p. 107245, Jul. 2020, https://doi.org/10.1016/j.patcog.2020.107245.
T. M. Ghazal et al., “Performances of K-Means Clustering Algorithm with Different Distance Metrics,” Intell. Autom. Soft Comput., vol. 29, no. 3, pp. 735–742, 2021, https://doi.org/10.32604/iasc.2021.019067.
D. M. SAPUTRA, D. SAPUTRA, and L. D. OSWARI, “Effect of Distance Metrics in Determining K-Value in K-Means Clustering Using Elbow and Silhouette Method,” in Proceedings of the Sriwijaya International Conference on Information Technology and Its Applications (SICONIAN 2019), 2020. https://doi.org/10.2991/aisr.k.200424.051.
I. H. Sarker, “Machine Learning: Algorithms, Real-World Applications and Research Directions,” SN Comput. Sci., vol. 2, no. 3, p. 160, May 2021, https://doi.org/10.1007/s42979-021-00592-x.
A. Chattopadhyay, P. Hassanzadeh, and S. Pasha, “Predicting clustered weather patterns: A test case for applications of convolutional neural networks to spatio-temporal climate data,” Sci. Rep., vol. 10, no. 1, p. 1317, Jan. 2020, https://doi.org/10.1038/s41598-020-57897-9.
A. A. M. Ahmed, M. H. Ahmed, S. K. Saha, O. Ahmed, and A. Sutradhar, “Optimization algorithms as training approach with hybrid deep learning methods to develop an ultraviolet index forecasting model,” Stoch. Environ. Res. Risk Assess., vol. 36, no. 10, pp. 3011–3039, Oct. 2022, https://doi.org/10.1007/s00477-022-02177-3
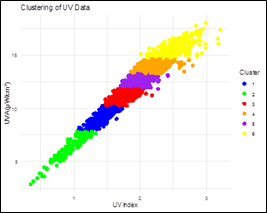
Downloads
Published
Issue
Section
License
Copyright (c) 2023 Edy Ervianto, Noveri Lysbetti Marpaung, Abu Yazid Raisal, Sakti Hutabarat, Rohana Hassan, Ruben Cornelius Siagian, Nurhalim, Rahyul Amri

This work is licensed under a Creative Commons Attribution-ShareAlike 4.0 International License.
Authors who publish in IRiP agree to the following terms: Authors retain copyright and grant the IRiP right of first publication with the work simultaneously licensed under a Creative Commons Attribution License (CC BY-SA 4.0) that allows others to share (copy and redistribute the material in any medium or format) and adapt (remix, transform, and build upon the material) the work for any purpose, even commercially with an acknowledgment of the work's authorship and initial publication in IRiP. Authors are able to enter into separate, additional contractual arrangements for the non-exclusive distribution of the journal's published version of the work (e.g., post it to an institutional repository or publish it in a book), with an acknowledgment of its initial publication in IRiP. Authors are permitted and encouraged to post their work online (e.g., in institutional repositories or on their website) prior to and during the submission process, as it can lead to productive exchanges, as well as earlier and greater citation of published work (See The Effect of Open Access).