Early Detection of Disease in Chicks Using CNN on Bangkok Chicken Health
DOI:
https://doi.org/10.12928/biste.v6i2.10245Keywords:
Bangkok Chicken, CNN, Early Detection of Disease, Identification, FarmAbstract
Bangkok Chicken (Gallus Gallus Domesticus) is a type of chicken in Indonesia that has a high source of protein and supports the community's economy. The growth and development phase of chicks is a critical period because chicks are very vulnerable to attacks by infectious and non-infectious diseases. These diseases can cause high mortality rates and cause significant economic losses for farmers. This study aimed to investigate the potential for using CNN technology in the early detection of disease in Bangkok chicks in the Ponorogo district. As an artificial neural network, CNN can recognize patterns in visual data with high accuracy. The use of CNN technology in the agricultural sector, including animal husbandry, has shown promising results in supporting early disease detection systems in livestock. This study aims to investigate the potential of using CNN technology in the early detection of disease in Bangkok chicks in the Ponorogo district. By processing visual data from chicken images, CNN will be trained to identify early signs of disease in chicks. The result of this research is that this research can help maintain the availability and security of animal food supplies, which is an essential component of overall food security. In addition, by reducing losses caused by disease, this research can contribute to sustainable agriculture by ensuring the continuation of stable and sustainable animal food production.
References
J. Marisa and S. A. Sitepu, “Profit analysis of broiler chicken business in Beringin Village, STM Hilir District, Deli Serdang Regency,” In IOP Conference Series: Earth and Environmental Science, vol. 287, no. 1, p. 012037, 2019, https://doi.org/10.1088/1755-1315/287/1/012037.
V. Mujyambere, K. Adomako, S. O. Olympio, M. Ntawubizi, L. Nyinawamwiza, J. Mahoro, and A. Conroy, “Local chickens in East African region: Their production and potential,” Poultry Science, vol. 101, no. 1, p. 101547, 2022, https://doi.org/10.1016/j.psj.2021.101547.
S. Y. Saif. Diseases of poultry. John Wiley & Sons. 2009. https://books.google.co.id/books?hl=id&lr=&id=ZVZ4whXoJa4C.
M. Suwannasri and B. Promphakping, “Sustainability of Smallholders in the Local Food System: A Study of Chicken Layer Farms in Nakhon Phanom Province, Northeast Thailand,” Sustainability, vol. 14, no. 10, p. 5997, 2022, https://doi.org/10.3390/su14105997.
Y. Gulzar, Y. Hamid, A. B. Soomro, A. A. Alwan, and L. Journaux, “A convolution neural network-based seed classification system,” Symmetry, vol. 12, no. 12, p. 2018, 2020, https://doi.org/10.3390/sym12122018.
X. Wang, W. Zhang, X. Wu, L. Xiao, Y. Qian, and Z. Fang, “Real-time vehicle type classification with deep convolutional neural networks,” Journal of Real-Time Image Processing, vol. 16, pp. 5-14, 2019, https://doi.org/10.1007/s11554-017-0712-5.
K. Simonyan and A. Zisserman, “Very deep convolutional networks for large-scale image recognition,” arXiv preprint arXiv:1409.1556, 2014, https://doi.org/10.48550/arXiv.1409.1556.
Y. Wang, C. Wei, H. Sun, and A. Qu, “Design of Intelligent Detection Platform for Wine Grape Pests and Diseases in Ningxia,” Plants, vol. 12, no. 1, p. 106, 2022, https://doi.org/10.3390/plants12010106.
M. Kholil, H. P. Waspada and R. Akhsani, "Classification of Infectious Diseases in Chickens Based on Feces Images Using Deep Learning," 2022 International Conference on Electrical and Information Technology (IEIT), pp. 362-365, 2022, https://doi.org/10.1109/IEIT56384.2022.9967850.
V. R. Sindhuja, M. Sobhana, V. Tejaswi and P. Durgesh, "Deep learning-based mobile application for nutrient deficiency identification of rice plants using ADAM Optimizer," 2022 IEEE Delhi Section Conference (DELCON), pp. 1-6, 2022, https://doi.org/10.1109/DELCON54057.2022.9752798.
D. Sitanggang, A. C. Sembiring, S. P. Tamba, W. Purba, V. Sihombing, S. Patricia, and I. Budiman, “Diagnosing chicken diseases using fuzzy Tsukamoto web-based expert system,” In IOP Conference Series: Materials Science and Engineering, vol. 505, no. 1, p. 012086, 2019, https://doi.org/10.1088/1757-899X/505/1/012086.
P. K. Sethy, N. K. Barpanda, A. K. Rath, and S. K. Behera, “Deep feature based rice leaf disease identification using support vector machine,” Computers and Electronics in Agriculture, vol. 175, p. 105527, 2020, https://doi.org/10.1016/j.compag.2020.105527.
I. S. Purba et al., “Accuracy Level of Backpropagation Algorithm to Predict Livestock Population of Simalungun Regency in Indonesia,” In Journal of Physics: Conference Series, vol. 1255, no. 1, p. 012014, 2019, https://doi.org/10.1088/1742-6596/1255/1/012014.
S. Widiyanto, R. Fitrianto and D. T. Wardani, "Implementation of Convolutional Neural Network Method for Classification of Diseases in Tomato Leaves," 2019 Fourth International Conference on Informatics and Computing (ICIC), pp. 1-5, 2019, https://doi.org/10.1109/ICIC47613.2019.8985909.
J. Dong, B. Lu, K. He, B. Li, B. Zhao, and X. Tang, “Assessment of hatching properties for identifying multiple duck eggs on the hatching tray using machine vision technique,” Computers and Electronics in Agriculture, vol. 184, p. 106076, 2021, https://doi.org/10.1016/j.compag.2021.106076.
T. Kattenborn, J. Leitloff, F. Schiefer, and S. Hinz, “Review on Convolutional Neural Networks (CNN) in vegetation remote sensing,” ISPRS journal of photogrammetry and remote sensing, vol. 173, pp. 24-49, 2021, https://doi.org/10.1016/j.isprsjprs.2020.12.010.
X. Wu, X. Liang, Y. Wang, B. Wu, and J. Sun, “Non-destructive techniques for the analysis and evaluation of meat quality and safety: A review,” Foods, vol. 11, no. 22, p. 3713, 2022, https://doi.org/10.3390/foods11223713.
R. P. Sidik and E. Contessa Djamal, "Face Mask Detection using Convolutional Neural Network," 2021 4th International Conference of Computer and Informatics Engineering (IC2IE), pp. 85-89, 2021, https://doi.org/10.1109/IC2IE53219.2021.9649065.
J. Jiao, M. Zhao, J. Lin, and K. Liang, “A comprehensive review on convolutional neural network in machine fault diagnosis,” Neurocomputing, vol. 417, pp. 36-63, 2020, https://doi.org/10.1016/j.neucom.2020.07.088.
Sakshi, C. Sharma, S. Sharma, T. Sharma and S. Gochhait, "Green Intelligence: A Sequential CNN Odyssey in Mustard Leaf Disease Detection," 2024 ASU International Conference in Emerging Technologies for Sustainability and Intelligent Systems (ICETSIS), Manama, Bahrain, 2024, pp. 877-882, 2024, https://doi.org/10.1109/ICETSIS61505.2024.10459509.
N. J S, K. Tharun and S. G. Sravya, "Deep Learning Approaches To Image-Based Species Identification," 2024 International Conference on Integrated Circuits and Communication Systems (ICICACS), pp. 1-7, 2024, https://doi.org/10.1109/ICICACS60521.2024.10498423.
H. C. Reis and V. Turk, “COVID-DSNet: A novel deep convolutional neural network for detection of coronavirus (SARS-CoV-2) cases from CT and Chest X-Ray images,” Artificial Intelligence in Medicine, vol. 134, p. 102427, 2022, https://doi.org/10.1016/j.artmed.2022.102427.
D. Bhatt et al., “CNN variants for computer vision: History, architecture, application, challenges and future scope,” Electronics, vol. 10, no. 20, p. 2470, 2021, https://doi.org/10.3390/electronics10202470.
A. W. Harley, A. Ufkes and K. G. Derpanis, "Evaluation of deep convolutional nets for document image classification and retrieval," 2015 13th International Conference on Document Analysis and Recognition (ICDAR), Tunis, Tunisia, 2015, pp. 991-995, 2015, https://doi.org/10.1109/ICDAR.2015.7333910.
Z. Li, F. Liu, W. Yang, S. Peng and J. Zhou, "A Survey of Convolutional Neural Networks: Analysis, Applications, and Prospects," in IEEE Transactions on Neural Networks and Learning Systems, vol. 33, no. 12, pp. 6999-7019, Dec. 2022, https://doi.org/10.1109/TNNLS.2021.3084827.
J. F. Gao et al., “Characterization of the mitochondrial genome of Tetrameres grusi and insights into the phylogeny of Spirurina,” International Journal for Parasitology: Parasites and Wildlife, vol. 17, pp. 35-42, 2022, https://doi.org/10.1016/j.ijppaw.2021.12.001.
R. Kanabarkar and M. Anandhalli, "Performance Analysis of Convolutional Neural Network for Image Classification," 2022 International Conference on Futuristic Technologies (INCOFT), pp. 1-4, 2022, https://doi.org/10.1109/INCOFT55651.2022.10094394.
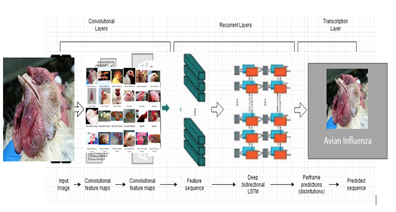
Downloads
Published
How to Cite
Issue
Section
License
Copyright (c) 2024 Agung Dwicahyo, Ilham Mufandi, Agustin Rani Nurfadila, Much. Taufik Ardani, Ubaid Dzilhilmi

This work is licensed under a Creative Commons Attribution-ShareAlike 4.0 International License.
Authors who publish with this journal agree to the following terms:
- Authors retain copyright and grant the journal right of first publication with the work simultaneously licensed under a Creative Commons Attribution License that allows others to share the work with an acknowledgment of the work's authorship and initial publication in this journal.
- Authors are able to enter into separate, additional contractual arrangements for the non-exclusive distribution of the journal's published version of the work (e.g., post it to an institutional repository or publish it in a book), with an acknowledgment of its initial publication in this journal.
- Authors are permitted and encouraged to post their work online (e.g., in institutional repositories or on their website) prior to and during the submission process, as it can lead to productive exchanges, as well as earlier and greater citation of published work (See The Effect of Open Access).
This journal is licensed under a Creative Commons Attribution-ShareAlike 4.0 International License.