Estimation of Flores Sea Aftershock Rupture Data Based on AI
DOI:
https://doi.org/10.12928/irip.v6i1.6705Keywords:
Aftershock, Flores Sea Earthquake, Machine Learning, Classification Algorithm, Regression AlgorithmAbstract
The earthquake catalog notes that there have been earthquakes with Mw > 7 that hit the Flores area, three of which occurred in the Flores Sea in 1992, 2015, and 2021. Revealed that the seismic activity of Eastern Indonesia is thought to be influenced by the isolated thrust fault segment of the island of Flores and the island of Wetar. The study of the rising fault segment on Flores Island and Wetar Island helps in further understanding the fault behavior, earthquake pattern, and seismic risk in the Flores Sea region. In earthquakes with giant magneto, an aftershock can occur due to the interaction of ground movements. This research analyzes and compares the data from the evaluation of the classification algorithm and the regression algorithm. The initial stages of this research include requesting IRIS DMC Web Service data. The data is then subjected to a cleaning process to obtain the expected feature extraction. The next stage is to perform the clustering process. This stage is carried out to label dependent data by adding new features as data clusters. The following procedure divides the validation value, which consists of training and test data. The estimation results show that the classification algorithm's evaluation value is better than that of the regression algorithm. The evaluation value of several algorithms indicates this, with an accuracy rate between 80% and 100%.
References
A. Jufriansah, A. Khusnani, Y. Pramudya, and M. Afriyanto, “Comparison of Aftershock Behavior of the Flores Sea 12 December 1992 and 14 December 2021,” J. Phys. Theor. Appl., vol. 7, no. 1, pp. 65–74, 2023.
I. R. Pranantyo, P. Cummins, J. Griffin, G. Davies, and H. Latief, “Modelling of Historical Tsunami in Eastern Indonesia: 1674 Ambon and 1992 Flores Case Studies,” in International Symposium on Earth Hazard and Disaster Mitigation (ISEDM) 2016: The 6th Annual Symposium on Earthquake and Related Geohazard Research for Disaster Risk Reduction, 2017, p. 090005, https://doi.org/10.1063/1.4987104.
A. Anggraini and E. Mardhatillah, “Perubahan Stress Statis Gempa Utama dan Asosiasi Distribusi Gempa Susulan: Studi Kasus Gempa Palu Mw 7,5 28 September 2018,” J. Fis. Indones., vol. 24, no. 1, p. 38, Jun. 2020, https://doi.org/10.22146/jfi.v24i1.53533.
B. J. Santosa, “Estimation of the Source Parameters of the Flores Earthquake and Their Correlation to Aftershocks,” IPTEK J. Eng., vol. 5, no. 1, pp. 14–17, May 2019, https://doi.org/10.12962/joe.v5i1.5021.
E. Mardhatillah, A. Anggraini, and M. Nukman, “Tinjauan Perubahan Stress Coulomb Ko-Seismik Pada Sekuens Gempa Palu M 7,5 28 September 2018,” J. Fis. Indones., vol. 24, no. 3, p. 175, Dec. 2020, https://doi.org/10.22146/jfi.v24i3.58237.
S. Baranov, C. Narteau, and P. Shebalin, “Modeling and Prediction of Aftershock Activity,” Surv. Geophys., vol. 43, pp. 437–481, Apr. 2022, https://doi.org/10.1007/s10712-022-09698-0.
R. P. Felix, J. A. Hubbard, K. E. Bradley, K. H. Lythgoe, L. Li, and A. D. Switzer, “Tsunami Hazard in Lombok and Bali, Indonesia, Due to the Flores Back-arc Thrust,” Nat. Hazards Earth Syst. Sci., vol. 22, no. 5, pp. 1665–1682, May 2022, https://doi.org/10.5194/nhess-22-1665-2022.
J. Schmidt, M. R. G. Marques, S. Botti, and M. A. L. Marques, “Recent Advances and Applications of Machine Learning in Solid-State Materials Science,” npj Comput. Mater., vol. 5, no. 1, p. 83, Aug. 2019, https://doi.org/10.1038/s41524-019-0221-0.
M. Wieland, W. Liu, and F. Yamazaki, “Learning Change from Synthetic Aperture Radar Images: Performance Evaluation of a Support Vector Machine to Detect Earthquake and Tsunami-Induced Changes,” Remote Sens., vol. 8, no. 10, p. 792, Sep. 2016, https://doi.org/10.3390/rs8100792.
M. Syifa, P. Kadavi, and C.-W. Lee, “An Artificial Intelligence Application for Post-Earthquake Damage Mapping in Palu, Central Sulawesi, Indonesia,” Sensors, vol. 19, no. 3, p. 542, Jan. 2019, https://doi.org/10.3390/s19030542.
M. H. Al Banna et al., “Application of Artificial Intelligence in Predicting Earthquakes: State-of-the-Art and Future Challenges,” IEEE Access, vol. 8, pp. 192880–192923, 2020, https://doi.org/10.1109/ACCESS.2020.3029859.
Q. Kong, D. T. Trugman, Z. E. Ross, M. J. Bianco, B. J. Meade, and P. Gerstoft, “Machine Learning in Seismology: Turning Data into Insights,” Seismol. Res. Lett., vol. 90, no. 1, pp. 3–14, Jan. 2019, https://doi.org/10.1785/0220180259.
F. Aden‐Antoniów, W. B. Frank, and L. Seydoux, “An Adaptable Random Forest Model for the Declustering of Earthquake Catalogs,” J. Geophys. Res. Solid Earth, vol. 127, no. 2, p. e2021JB023254, Feb. 2022, https://doi.org/10.1029/2021JB023254.
Y. Zhang and K.-V. Yuen, “Review of Artificial Intelligence-based Bridge Damage Detection,” Adv. Mech. Eng., vol. 14, no. 9, pp. 1–21, Sep. 2022, https://doi.org/10.1177/16878132221122770.
S. Liu, Y. Jiang, M. Li, J. Xin, and L. Peng, “Long Period Ground Motion Simulation and Its Application to the Seismic Design of High-Rise Buildings,” Soil Dyn. Earthq. Eng., vol. 143, p. 106619, Apr. 2021, https://doi.org/10.1016/j.soildyn.2021.106619.
Y. Xie, M. Ebad Sichani, J. E. Padgett, and R. DesRoches, “The Promise of Implementing Machine Learning in Earthquake Engineering: A State-of-the-art Review,” Earthq. Spectra, vol. 36, no. 4, pp. 1769–1801, Nov. 2020, https://doi.org/10.1177/8755293020919419.
T. S. Bressan, M. Kehl de Souza, T. J. Girelli, and F. C. Junior, “Evaluation of Machine Learning Methods for Lithology Classification Using Geophysical Data,” Comput. Geosci., vol. 139, p. 104475, Jun. 2020, https://doi.org/10.1016/j.cageo.2020.104475.
Y. Ao, H. Li, L. Zhu, S. Ali, and Z. Yang, “Identifying Channel Sand-Body from Multiple Seismic Attributes with an Improved Random Forest Algorithm,” J. Pet. Sci. Eng., vol. 173, pp. 781–792, Feb. 2019, https://doi.org/10.1016/j.petrol.2018.10.048.
R. Jena and B. Pradhan, “Integrated ANN-cross-validation and AHP-TOPSIS Model to Improve Earthquake Risk Assessment,” Int. J. Disaster Risk Reduct., vol. 50, p. 101723, Nov. 2020, https://doi.org/10.1016/j.ijdrr.2020.101723.
Y. Xia, C. Liu, Y. Li, and N. Liu, “A Boosted Decision Tree Approach Using Bayesian Hyper-Parameter Optimization for Credit Scoring,” Expert Syst. Appl., vol. 78, pp. 225–241, Jul. 2017, https://doi.org/10.1016/j.eswa.2017.02.017.
L. Yang and A. Shami, “On Hyperparameter Optimization of Machine Learning Algorithms: Theory and Practice,” Neurocomputing, vol. 415, pp. 295–316, Nov. 2020, https://doi.org/10.1016/j.neucom.2020.07.061.
M. A. Sebtosheikh and A. Salehi, “Lithology Prediction by Support Vector Classifiers Using Inverted Seismic Attributes Data and Petrophysical Logs as A New Approach and Investigation of Training Data Set Size Effect on Its Performance in A Heterogeneous Carbonate Reservoir,” J. Pet. Sci. Eng., vol. 134, pp. 143–149, Oct. 2015, https://doi.org/10.1016/j.petrol.2015.08.001.
I. Laory, T. N. Trinh, I. F. C. Smith, and J. M. W. Brownjohn, “Methodologies for Predicting Natural Frequency Variation af A Suspension Bridge,” Eng. Struct., vol. 80, pp. 211–221, Dec. 2014, https://doi.org/10.1016/j.engstruct.2014.09.001.
D. Tao, Q. Ma, S. Li, Z. Xie, D. Lin, and S. Li, “Support Vector Regression for the Relationships between Ground Motion Parameters and Macroseismic Intensity in the Sichuan–Yunnan Region,” Appl. Sci., vol. 10, no. 9, p. 3086, Apr. 2020, https://doi.org/10.3390/app10093086.
T. Sakaki, M. Okazaki, and Y. Matsuo, “Earthquake Shakes Twitter Users,” in Proceedings of the 19th international conference on World wide web, Apr. 2010, pp. 851–860, https://doi.org/10.1145/1772690.1772777.
S. Stramondo, C. Bignami, M. Chini, N. Pierdicca, and A. Tertulliani, “Satellite Radar and Optical Remote Sensing for Earthquake Damage Detection: Results from Different Case Studies,” Int. J. Remote Sens., vol. 27, no. 20, pp. 4433–4447, Oct. 2006, https://doi.org/10.1080/01431160600675895.
D. Pasten, G. Saravia, E. E. Vogel, and A. Posadas, “Information Theory and Earthquakes: Depth Propagation Seismicity In Northern Chile,” Chaos, Solitons & Fractals, vol. 165, p. 112874, Dec. 2022, https://doi.org/10.1016/j.chaos.2022.112874.
P. Zhou, H. Yang, B. Wang, and J. Zhuang, “Seismological Investigations of Induced Earthquakes Near the Hutubi Underground Gas Storage Facility,” J. Geophys. Res. Solid Earth, vol. 124, no. 8, pp. 8753–8770, Aug. 2019, https://doi.org/10.1029/2019JB017360.
P. Yariyan, H. Zabihi, I. D. Wolf, M. Karami, and S. Amiriyan, “Earthquake Risk Assessment Using an Integrated Fuzzy Analytic Hierarchy Process with Artificial Neural Networks based on GIS: A case Study of Sanandaj in Iran,” Int. J. Disaster Risk Reduct., vol. 50, p. 101705, Nov. 2020, https://doi.org/10.1016/j.ijdrr.2020.101705.
J. Roiz-Pagador, A. Chacon-Maldonado, R. Ruiz, and G. Asencio-Cortes, “Earthquake Prediction in California Using Feature Selection Techniques,” in 16th International Conference on Soft Computing Models in Industrial and Environmental Applications (SOCO 2021), 2022, pp. 728–738, https://doi.org/10.1007/978-3-030-87869-6_69.
T.-Y. Hsu et al., “Rapid On-Site Peak Ground Acceleration Estimation Based on Support Vector Regression and P-Wave Features in Taiwan,” Soil Dyn. Earthq. Eng., vol. 49, pp. 210–217, Jun. 2013, https://doi.org/10.1016/j.soildyn.2013.03.001.
T. Zhao, V. Jayaram, A. Roy, and K. J. Marfurt, “A Comparison of Classification Techniques for Seismic Facies Recognition,” Interpretation, vol. 3, no. 4, pp. SAE29–SAE58, Nov. 2015, https://doi.org/10.1190/INT-2015-0044.1.
F. Soleimani, “Analytical Seismic Performance and Sensitivity Evaluation of Bridges Based on Random Decision Forest Framework,” Structures, vol. 32, pp. 329–341, Aug. 2021, https://doi.org/10.1016/j.istruc.2021.02.049.
X. Fan et al., “Earthquake‐Induced Chains of Geologic Hazards: Patterns, Mechanisms, and Impacts,” Rev. Geophys., vol. 57, no. 2, pp. 421–503, Jun. 2019, https://doi.org/10.1029/2018RG000626.
S. Mangalathu, H. Sun, C. C. Nweke, Z. Yi, and H. V. Burton, “Classifying Earthquake Damage to Buildings Using Machine Learning,” Earthq. Spectra, vol. 36, no. 1, pp. 183–208, Feb. 2020, https://doi.org/10.1177/8755293019878137.
T. Kim, J. Song, and O.-S. Kwon, “Probabilistic Evaluation of Seismic Responses Using Deep Learning Method,” Struct. Saf., vol. 84, p. 101913, May 2020, https://doi.org/10.1016/j.strusafe.2019.101913.
S. Bouktif, A. Fiaz, A. Ouni, and M. Serhani, “Optimal Deep Learning LSTM Model for Electric Load Forecasting using Feature Selection and Genetic Algorithm: Comparison with Machine Learning Approaches †,” Energies, vol. 11, no. 7, p. 1636, Jun. 2018, https://doi.org/10.3390/en11071636.
S. Rajkumari, K. Thakkar, and H. Goyal, “Fragility Analysis of Structures Subjected to Seismic Excitation: A State-of-the-art Review,” Structures, vol. 40, pp. 303–316, Jun. 2022, https://doi.org/10.1016/j.istruc.2022.04.023.
R. J. Geller, “Earthquake Prediction: A Critical Review,” Geophys. J. Int., vol. 131, no. 3, pp. 425–450, Dec. 1997, https://doi.org/10.1111/j.1365-246X.1997.tb06588.x.
S. Uyeda, “The VAN Method of Short-Term Earthquake Prediction,” INCEDE Newslett, vol. 5, no. 4, pp. 2–4, 1997, [Online]. Available: https://www.sems-tokaiuniv.jp/old/eprc/res/incede/incede2.html.
L. Sevgi, “A Critical Review on Electromagnetic Precursors and Earthquake Prediction,” Turkish J. Electr. Eng. Comput. Sci., vol. 15, no. 1, pp. 1–15, 2007, [Online]. Available: https://journals.tubitak.gov.tr/elektrik/vol15/iss1/1.
F. Huang et al., “Studies on Earthquake Precursors in China: A Review for Recent 50 Years,” Geod. Geodyn., vol. 8, no. 1, pp. 1–12, Jan. 2017, https://doi.org/10.1016/j.geog.2016.12.002.
A. Mignan and M. Broccardo, “Neural Network Applications in Earthquake Prediction (1994–2019): Meta-Analytic and Statistical Insights on Their Limitations,” Seismol. Res. Lett., vol. 91, no. 4, pp. 2330–2342, Jul. 2020, https://doi.org/10.1785/0220200021.
A. Mignan and M. Broccardo, “One Neuron Versus Deep Learning in Aftershock Prediction,” Nature, vol. 574, no. 7776, pp. E1–E3, Oct. 2019, https://doi.org/10.1038/s41586-019-1582-8.
A. Mignan and M. Broccardo, “A Deeper Look into ‘Deep Learning of Aftershock Patterns Following Large Earthquakes’: Illustrating First Principles in Neural Network Physical Interpretability,” in Advances in Computational Intelligence. IWANN 2019, 2019, pp. 3–14, https://doi.org/10.1007/978-3-030-20521-8_1.
S. Karimzadeh, M. Matsuoka, J. Kuang, and L. Ge, “Spatial Prediction of Aftershocks Triggered by a Major Earthquake: A Binary Machine Learning Perspective,” ISPRS Int. J. Geo-Information, vol. 8, no. 10, p. 462, Oct. 2019, https://doi.org/10.3390/ijgi8100462.
L. Handayani, “Seismic Hazard Analysis of Maumere, Flores: a Review of the Earthquake Sources,” 2020, https://doi.org/10.4108/eai.12-10-2019.2296247.
R. Maneno, B. J. Sentosa, and G. Rachman, “Relocation of Earthquake Hypocenter in the Flores Region Using Hypo71,” IPTEK J. Eng., vol. 5, no. 2, pp. 33–37, May 2019, https://doi.org/10.12962/j23378557.v5i2.a5024.
H. Kurnio, T. Naibaho, and C. Purwanto, “Review of Submarine Landslides in the Eastern Indonesia Region,” Bull. Mar. Geol., vol. 34, no. 2, pp. 63–76, Dec. 2019, https://doi.org/10.32693/bomg.34.2.2019.618.
I. R. Pranantyo, M. Heidarzadeh, and P. R. Cummins, “Complex Tsunami Hazards in Eastern Indonesia from Seismic and Non-Seismic Sources: Deterministic Modelling Based on Historical and Modern Data,” Geosci. Lett., vol. 8, no. 1, p. 20, Dec. 2021, https://doi.org/10.1186/s40562-021-00190-y.
P. Supendi et al., “Relocated Aftershocks and Background Seismicity in Eastern Indonesia Shed Light on the 2018 Lombok and Palu Earthquake Sequences,” Geophys. J. Int., vol. 221, no. 3, pp. 1845–1855, Jun. 2020, https://doi.org/10.1093/gji/ggaa118.
A. Jufriansah, Y. Pramudya, A. Khusnani, and S. Saputra, “Analysis of Earthquake Activity in Indonesia by Clustering Method,” J. Phys. Theor. Appl., vol. 5, no. 2, p. 92, Sep. 2021, https://doi.org/10.20961/jphystheor-appl.v5i2.59133.
A. Chantaramanee, K. Nakagawa, K. Yoshimi, A. Nakane, K. Yamaguchi, and H. Tohara, “Comparison of Tongue Characteristics Classified According to Ultrasonographic Features Using a K-Means Clustering Algorithm,” Diagnostics, vol. 12, no. 2, p. 264, Jan. 2022, https://doi.org/10.3390/diagnostics12020264.
C. Yuan and H. Yang, “Research on K-Value Selection Method of K-Means Clustering Algorithm,” J, vol. 2, no. 2, pp. 226–235, Jun. 2019, https://doi.org/10.3390/j2020016.
C. Jiang, X. Wei, X. Cui, and D. You, “Application of Support Vector Machine to Synthetic Earthquake Prediction,” Earthq. Sci., vol. 22, no. 3, pp. 315–320, Jun. 2009, https://doi.org/10.1007/s11589-009-0315-8.
W. Astuti, R. Akmeliawati, W. Sediono, and M. J. E. Salami, “Hybrid Technique Using Singular Value Decomposition (SVD) and Support Vector Machine (SVM) Approach for Earthquake Prediction,” IEEE J. Sel. Top. Appl. Earth Obs. Remote Sens., vol. 7, no. 5, pp. 1719–1728, May 2014, https://doi.org/10.1109/JSTARS.2014.2321972.
S. Marhain, A. N. Ahmed, M. A. Murti, P. Kumar, and A. El-Shafie, “Investigating the Application of Artificial Intelligence for Earthquake Prediction In Terengganu,” Nat. Hazards, vol. 108, no. 1, pp. 977–999, Aug. 2021, https://doi.org/10.1007/s11069-021-04716-7.
Y. Essam, P. Kumar, A. N. Ahmed, M. A. Murti, and A. El-Shafie, “Exploring the Reliability of Different Artificial Intelligence Techniques In Predicting Earthquake for Malaysia,” Soil Dyn. Earthq. Eng., vol. 147, p. 106826, Aug. 2021, https://doi.org/10.1016/j.soildyn.2021.106826.
Z. Huang, C. Yang, X. Zhou, and S. Yang, “Energy Consumption Forecasting for the Nonferrous Metallurgy Industry Using Hybrid Support Vector Regression with an Adaptive State Transition Algorithm,” Cognit. Comput., vol. 12, no. 2, pp. 357–368, Mar. 2020, https://doi.org/10.1007/s12559-019-09644-0.
I. Aljarah, A. M. Al-Zoubi, H. Faris, M. A. Hassonah, S. Mirjalili, and H. Saadeh, “Simultaneous Feature Selection and Support Vector Machine Optimization Using the Grasshopper Optimization Algorithm,” Cognit. Comput., vol. 10, no. 3, pp. 478–495, Jun. 2018, https://doi.org/10.1007/s12559-017-9542-9.
A. Lulli, L. Oneto, and D. Anguita, “Mining Big Data with Random Forests,” Cognit. Comput., vol. 11, no. 2, pp. 294–316, Apr. 2019, https://doi.org/10.1007/s12559-018-9615-4.
M. N. Shodiq, D. H. Kusuma, M. G. Rifqi, A. R. Barakbah, and T. Harsono, “Neural Network for Earthquake Prediction Based on Automatic Clustering in Indonesia,” JOIV Int. J. Informatics Vis., vol. 2, no. 1, p. 37, Feb. 2018, https://doi.org/10.30630/joiv.2.1.106.
M. N. Shodiq, D. H. Kusuma, M. G. Rifqi, A. R. Barakbah, and T. Harsono, “Spatial Analisys of Magnitude Distribution for Earthquake Prediction Using Neural Network Based on Automatic Clustering In Indonesia,” in 2017 International Electronics Symposium on Knowledge Creation and Intelligent Computing (IES-KCIC), Sep. 2017, pp. 246–251, https://doi.org/10.1109/KCIC.2017.8228594.
A. S. N. Alarifi, N. S. N. Alarifi, and S. Al-Humidan, “Earthquakes Magnitude Predication Using Artificial Neural Network in Northern Red Sea Area,” J. King Saud Univ. - Sci., vol. 24, no. 4, pp. 301–313, Oct. 2012, https://doi.org/10.1016/j.jksus.2011.05.002.
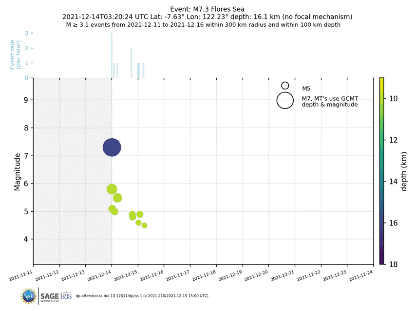
Downloads
Published
Issue
Section
License
Copyright (c) 2023 Adi Jufriansah, Azmi Khusnani, Yudhiakto Pramudya, Mulya Afriyanto

This work is licensed under a Creative Commons Attribution-ShareAlike 4.0 International License.
Authors who publish in IRiP agree to the following terms: Authors retain copyright and grant the IRiP right of first publication with the work simultaneously licensed under a Creative Commons Attribution License (CC BY-SA 4.0) that allows others to share (copy and redistribute the material in any medium or format) and adapt (remix, transform, and build upon the material) the work for any purpose, even commercially with an acknowledgment of the work's authorship and initial publication in IRiP. Authors are able to enter into separate, additional contractual arrangements for the non-exclusive distribution of the journal's published version of the work (e.g., post it to an institutional repository or publish it in a book), with an acknowledgment of its initial publication in IRiP. Authors are permitted and encouraged to post their work online (e.g., in institutional repositories or on their website) prior to and during the submission process, as it can lead to productive exchanges, as well as earlier and greater citation of published work (See The Effect of Open Access).