Long Short-Term Memory on Bitcoin Price Forecasting
DOI:
https://doi.org/10.12928/mf.v3i1.3857Keywords:
Bitcoin, LSTM, Forecasting, MAPEAbstract
In modern times, many people rely on sophisticated technology to meet their needs. Already many technologies today can replace the role and function of society in the field of investment. There are many ways to fulfill the lives of these people, such as Bitcoin investment. Bitcoin is a digital asset that only exists in digital form by means of peer-to-peer work. To maximize profits, it is necessary to forecast Bitcoin prices when it will go up or down. This study tries to address the changes in Bitcoin prices whether to go up or down the next day with an artificial neural network model. The editor used in this study is the LSTM method. The data used is the Bitcoin blockchain data, namely time-series data in a one-day period from 1 January 2018 to 31 May 2019. Obtained forecasting results in June 2019 for Bitcoin to rise slowly and an accuracy value of 97.5% based on MAPE with the first day worth $8901.50.
References
Aflahi, T. (2018). Tutorial Deep Learning untuk Pemula. Diambil kembali dari https://pendekardata.com/tutorial-deep-learning-untuk-pemula/
Andi. (2008, November). Melakukan Preprocessing Data. Diambil kembali dari https://andyku.wordpress.com/2008/11/21/melakukan-preprocessing-data/
CoinDesk. Bitcoin Price (BTC). Diambil kembali dari https://www.coindesk.com/price/bitcoin
CoinMarketCap. Cryptocurrencies. Diambil kembali dari https://coinmarketcap.com/
Dadang, W. (2018, Februari). Memahami Kecerdasan Buatan berupa Deep Learning dan Machine Learning. Diambil kembali dari https://warstek.com/2018/02/06/deepmachinelearning/
Olah, C. (2015, Agustus). Understanding LSTM Networks. Diambil kembali dari http://colah.github.io/posts/2015-08-Understanding-LSTMs/
Prijono, B. (2018, April). Pengenalan Long Short Term Memory (LSTM) dan Gated Recurrent Unit (GRU) – RNN Bagian 2. Diambil kembali dari https://indoml.com/2018/04/13/pengenalan-long-short-term-memory-lstm-dan-gated-recurrent-unit-gru-rnn-bagian-2/
Putra, M. W. (2018). Analisis dan implementasi Long Short Term Memory Neural Network untuk Prediksi Harga Bitcoin . e-Proceeding of Engineering.
Ryan, M. (2017). Pengenalan LSTM (Long Short Term Memory). Diambil kembali dari https://www.academia.edu/34438387/PENGENALAN_LSTM_LONG_SHORT_TERM_MEMORY_
Salwa, N., Tatsara, N. & dkk. (2018). Peramalan Harga Bitcoin Menggunakan Metode ARIMA (Autoregressive Integrated Moving Average). Journal of Data Analysis, 21-31.
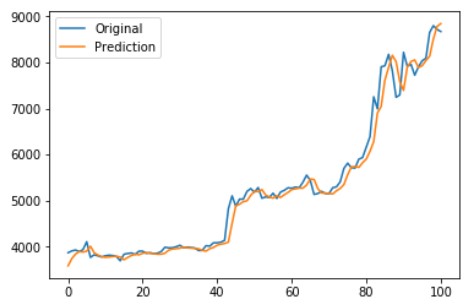
Downloads
Published
Issue
Section
License
Copyright (c) 2021 Tuti Purwaningsih, Gita Evi Kusumandari

This work is licensed under a Creative Commons Attribution-ShareAlike 4.0 International License.
Start from 2019 issues, authors who publish with JURNAL MOBILE AND FORENSICS agree to the following terms:
- Authors retain copyright and grant the journal right of first publication with the work simultaneously licensed under a Creative Commons Attribution License (CC BY-SA 4.0) that allows others to share the work with an acknowledgment of the work's authorship and initial publication in this journal.
- Authors are able to enter into separate, additional contractual arrangements for the non-exclusive distribution of the journal's published version of the work (e.g., post it to an institutional repository or publish it in a book), with an acknowledgment of its initial publication in this journal.
- Authors are permitted and encouraged to post their work online (e.g., in institutional repositories or on their website) prior to and during the submission process, as it can lead to productive exchanges, as well as earlier and greater citation of published work.
This work is licensed under a Creative Commons Attribution-ShareAlike 4.0 International License.