Prediksi Lama Studi Mahasiswa Menggunakan Naïve Bayes Berdasarkan Aspek Sosial Ekonomi Mahasiwa
DOI:
https://doi.org/10.12928/mf.v2i1.2137Keywords:
Prediksi Lama Studi, Data Mining, K-fold Cross Validation Naïve Bayes, MahasiswaAbstract
Perguruan tinggi merupakan satuan penyelenggara pendidikan tinggi sebagai tingkat lanjut jenjang pendidikan menengah di jalur pendidikan formal. Kualitas perguruan tinggi, khususnya perguruan tinggi di Indonesia diukur berdasarkan 9 standar utama. Salah satu aspek yang berpengaruh ialah mahasiswa dan lulusan. Ketepatan waktu studi mahasiswa adalah hal yang penting dalam perguruan tinggi. Ketepatan waktu mahasiswa dalam menyelesaikan studi menjadi salah satu penunjang penilaian kualitas perguruan tinggi. Metode Naïve Bayes dapat digunakan untuk memprediksi ketepatan lama studi. Klasifikasi Naïve Bayes dalam penelitian ini menggunakan beberapa variabel yang sangat erat kaitannya dalam menyelesaikan studi khususnya pada aspek sosial ekonomi mahasiswa. Adapun variable dari sisi sosial dan ekonomi tersebut diantaranya jenis kelamin, nilai IPK, tempat lahir, tipe sekolah, jumlah keikutsertaan organisasi, tingkat ekonomi, dan dukungan orang tua. Pada penelitian ini, metode Naïve Bayes diimplementasikan pada kasus prediksi lama studi mahasiswa menggunakan 200 data set. Hasil penelitian menunjukkan tingkat rata-rata akurasi sebesar 80,5% dengan menggunakan K-Fold Cross Validation diperoleh standar deviasi 3,02%.
Higher education is a higher education provider unit as an advanced level of secondary education in the formal education pathway. The quality of tertiary institutions, especially tertiary institutions in Indonesia, is measured according to 9 main standards. One influential aspect is students and graduates. Timeliness of student studies is important in higher education. Timeliness of students in completing their studies is one of the supports for assessing the quality of higher education. The Naïve Bayes method can be used to predict the accuracy of the study duration. Naïve Bayes classification in this study uses several variables that are very closely related in completing studies, especially on the social economic aspects of students. The social and economic variables include gender, GPA, birthplace, type of school, number of organizational participations, economic level, and parent support. In this study, the Naïve Bayes method is implemented in the case of prediction of student study duration using 200 data sets. The results showed an average level of accuracy of 80.5% using K-Fold Cross Validation obtained a standard deviation of 3.02%.
References
BAN-PT, "Instrumen Akreditasi Perguruan Tinggi," no. April, pp. 7-9, 2019.
I. B. A. Peling, I. N. Arnawan, I. P. A. Arthawan, and I. G. N. Janardana, "Implementation of Data Mining To Predict Period of Students Study Using Naïve Bayes Algorithm," Int. J. Eng. Emerg. Technol., vol. 2, no. 1, p. 53, 2017.
B. D. F. Kurniatullah and Y. T. C. Pramudi, "Estimation of Student's Graduation Using Multiple Linear Regression Method," J. Appl. Intell. Syst., vol. 2, no. 1, pp. 29-36, 2017.
M. I. Zulfa, A. Fadli, and Y. Ramadhani, "Classification model for graduation on time study using data mining techniques with SVM algorithm," AIP Conf. Proc., vol. 2094, no. April, 2019.
A. Kesumawati and D. T. Utari, "Predicting patterns of student graduation rates using Naïve bayes classifier and support vector machine Predicting Patterns of Student Graduation Rates Using Naïve Bayes Classifier and Support Vector Machine," vol. 060005, no. October 2018, 2021.
A. D. Herlambang, S. H. Wijoyo, and A. Rachmadi, "Intelligent Computing System to Predict Vocational High School Student Learning Achievement Using Naive Bayes Algorithm," vol. 4, no. 1, pp. 15-25, 2019.
A. Kasem, "Learning Analytics in Universiti Teknologi Brunei : Predicting Graduates Performance," 2018 Fourth Int. Conf. Adv. Comput. Commun. Autom., vol. 2017, no. 4, pp. 1-5, 2018.
F. Razaque et al., "Using naïve bayes algorithm to student's bachelor academic performances analysis," 4th IEEE Int. Conf. Eng. Technol. Appl. Sci. ICETAS 2017, vol. 2018-Janua, pp. 1-5, 2018.
O. Moscoso-zea, P. Saa, and S. Luján-mora, "Evaluation of algorithms to predict graduation rate in higher education institutions by applying educational data mining," Australas. J. Eng. Educ., vol. 00, no. 00, pp. 1-10, 2019.
S. Lolo and S. Ricambi, "AN ANALYSIS OF BAYESIAN ALGORITHM IN GRADUATES TO STUDY FURTHER INTO UNIVERSITY," pp. 871-879, 2019.
G. Wang, "Applying Customer Loyalty Classification with RFM and Naïve Bayes for Better Decision Making," 2019 Int. Semin. Appl. Technol. Inf. Commun., pp. 564-568, 2019.
Y. Ko, "How to use negative class information for Naive Bayes classification," Inf. Process. Manag., vol. 53, no. 6, pp. 1255-1268, 2017.
W. Astuti, "Kinerja Metode Naïve Bayes dalam Prediksi Lama Studi Mahasiswa Fakultas Ilmu Komputer," vol. 3, no. 2, pp. 107-111, 2018.
M. Sivasakthi, "Classification and prediction based data mining algorithms to predict students introductory programming performance," Proc. Int. Conf. Inven. Comput. Informatics, ICICI 2017, no. Icici, pp. 346-350, 2018.
J. Y. Hutapea, Y. T. Samuel, and H. Sitorus, "a Comparison Of Accuracy Between Two Methods Naive Bayes Algorithm And Decision Tree-J48 To Predict The Stock Price Of Pt Astra Internasional Tbk Using Data From Indonesia Stock Exchange," Abstr. Proc. Int. Sch. Conf., vol. 7, no. 1, pp. 1244-1258, 2019.
T. Imandasari, E. Irawan, A. P. Windarto, and A. Wanto, "Algoritma Naive Bayes Dalam Klasifikasi Lokasi Pembangunan Sumber Air," Pros. Semin. Nas. Ris. Inf. Sci., vol. 1, no. November, p. 750, 2019.
A. P. Wibawa et al., "Naïve Bayes Classifier for Journal Quartile Classification," Int. J. Recent Contrib. from Eng. Sci. IT, vol. 7, no. 2, p. 91, 2019.
Y. A. Gerhana, I. Fallah, W. B. Zulfikar, D. S. Maylawati, and M. A. Ramdhani, "Comparison of naive Bayes classifier and C4.5 algorithms in predicting student study period," J. Phys. Conf. Ser., vol. 1280, no. 2, 2019.
K. J. Grimm, G. L. Mazza, and P. Davoudzadeh, "Model Selection in Finite Mixture Models: A k-Fold Cross-Validation Approach," Struct. Equ. Model., vol. 24, no. 2, pp. 246-256, 2017.
R. Valavi, J. Elith, J. J. Lahoz-Monfort, and G. Guillera-Arroita, "blockCV: An r package for generating spatially or environmentally separated folds for k-fold cross-validation of species distribution models," Methods Ecol. Evol., vol. 10, no. 2, pp. 225-232, 2019.
F. Tempola, M. Muhammad, and A. Khairan, "Perbandingan Klasifikasi Antara Knn Dan Naive Bayes Pada Penentuan Status Gunung Berapi Dengan K-Fold Cross Validation Comparison of Classification Between Knn and Naive Bayes At the Determination of the Volcanic Status With K-Fold Cross," J. Teknol. Inf. dan Ilmu Komput., vol. 5, no. 5, pp. 577-584, 2018.
B. Cahyono, "ANALISIS KETRAMPILAN BERFIKIR KRITIS DALAM," vol. 8, no. 1, pp. 50-64, 2017.
A. A. Rondiyah, N. E. Wardani, and K. Saddhono, "UNTUK MENINGKATKAN PENDIDIKAN KARAKTER KEBANGSAAN DI ERA MEA ( MASAYARAKAT EKONOMI ASEAN )," pp. 141-147, 2015.
T. Carmelia, S. Tiatri, E. Wijaya, and L. Belakang, "Akademik Dengan JOB Performance Pada Mahasiswa Aktif Organisasi Kemahasiswaan," pp. 184-197, 2017.
S. Haq, "Economic Education Analysis Journal," vol. 5, no. 3, pp. 1034-1045, 2016.
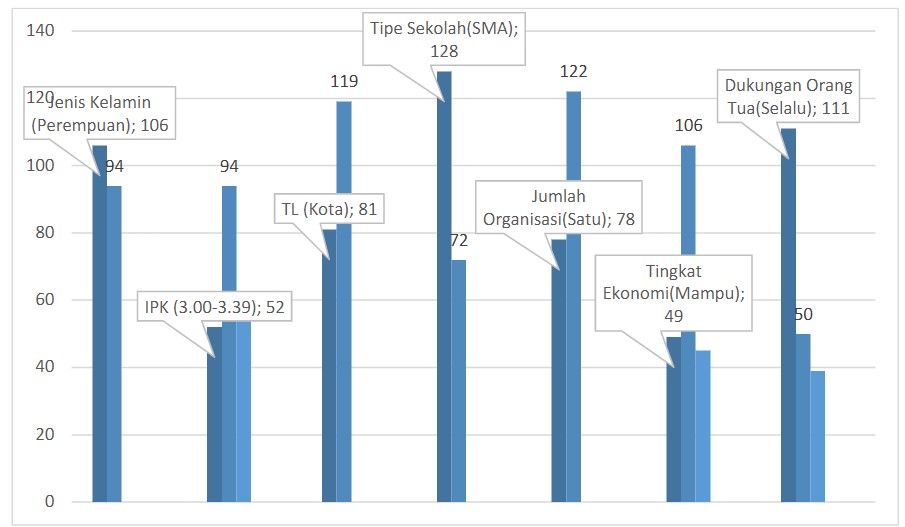
Downloads
Published
Issue
Section
License
Copyright (c) 2020 Desy Pratiwi Ika Putri, Desi Anggreani, Aji Prasetya Wibawa

This work is licensed under a Creative Commons Attribution-ShareAlike 4.0 International License.
Start from 2019 issues, authors who publish with JURNAL MOBILE AND FORENSICS agree to the following terms:
- Authors retain copyright and grant the journal right of first publication with the work simultaneously licensed under a Creative Commons Attribution License (CC BY-SA 4.0) that allows others to share the work with an acknowledgment of the work's authorship and initial publication in this journal.
- Authors are able to enter into separate, additional contractual arrangements for the non-exclusive distribution of the journal's published version of the work (e.g., post it to an institutional repository or publish it in a book), with an acknowledgment of its initial publication in this journal.
- Authors are permitted and encouraged to post their work online (e.g., in institutional repositories or on their website) prior to and during the submission process, as it can lead to productive exchanges, as well as earlier and greater citation of published work.
This work is licensed under a Creative Commons Attribution-ShareAlike 4.0 International License.