Classification of student abilities in reducing students’ drop-out rates
DOI:
https://doi.org/10.12928/bamme.v3i2.10040Keywords:
Education data mining, Reducing drop-out rates, Student ability classificationAbstract
The number of students in the mathematics education study program has declined over the past four years. Besides a few students enrolled in the first year, there are those who have had a temporary leave. These two things are necessary to identify the cause of study leave and need to analyze education data mining (EDM) to obtain a model of classification of students' abilities so that students can improve their achievement. The impact will be a decrease in the number of students undergoing the study. The subject of this study is a student of mathematics education study program from 2008 to 2018. Based on this data set, each student has information about gender, date of birth, home address, postal code, parent's name, secondary school origin, parent job, parent income cell phone number, graduation/drop-out status. The aim of the study is to identify an efficient model between a decision tree, a random forest and a neural network, based on the accuracy of 80 percent decision tree methods, 87 percent random forest methods and 78 percent neural networks.
References
Baek, C., & Doleck, T. (2021). Educational Data Mining versus Learning Analytics: A Review of Publications From 2015 to 2019. Interactive Learning Environments, 31(6), 3828–3850. https://doi.org/10.1080/10494820.2021.1943689
Berland, M., Baker, R. S., & Blikstein, P. (2014). Educational data mining and learning analytics: Applications to constructionist research. In Technology, Knowledge and Learning (Vol. 19, Issues 1–2, pp. 205–220). Kluwer Academic Publishers. https://doi.org/10.1007/s10758-014-9223-7
Bishop, C. M. (1995). Neural Networks for Pattern Recognition. Clarendo Press.
Bramer, M. (2020). Principles of Data Mining. Springer London. https://doi.org/10.1007/978-1-4471-7493-6
Cortez, P., & Silva, A. (2015). Using Data Mining to Predict Secondary School Student Performance.
Dreyfus, G. (2005). Neural Networks: Methodology and Applications. Springer-Verlag Berlin Heidelberg.
Ertel, W. (2017). Introduction to Artificial Intelligence (2nd ed.). Springer International Publishing AG. https://doi.org/10.1007/978-3-319-58487-4
Hwang, G. J., Xie, H., Wah, B. W., & Gašević, D. (2020). Vision, challenges, roles and research issues of Artificial Intelligence in Education. In Computers and Education: Artificial Intelligence (Vol. 1). Elsevier B.V. https://doi.org/10.1016/j.caeai.2020.100001
Jacob, J., Jha, K., Kotak, P., & Puthran, S. (2015). Educational Data Mining Techniques and their Applications. Proceedings of the 2015 International Conference on Green Computing and Internet of Things (ICGCIoT) 8-10 October 2015, Greater Noida, India : Venue: GCET, Greater Noida, Delhi, 1344–1348.
Jalota, C., & Agrawal, R. (2019). Analysis of Educational Data Mining using Classification. Proceedings of the International Conference on Machine Learning, Big Data, Cloud and Parallel Computing : Trends, Prespectives and Prospects : COMITCON-2019 : 14th-16th February, 2019, 243–247.
Larose, D. T. (2005). Discovering knowledge in data: An Introduction to Data Mining. John Wiley & Sons, Inc., Hoboken, New Jersey.
Lintas, A., Rovetta, S., Verschure, P. F. M. J., & Villa, A. E. P. (2017). Artificial Neural Networks and Machine Learning-ICANN 2017. 26th International Conference on Artificial Neural Networks Alghero, Italy,. https://doi.org/https://doi.org/10.1007/978-3-319-68612-7
Pal, S. (2012). Mining Educational Data to Reduce Dropout Rates of Engineering Students. International Journal of Information Engineering and Electronic Business, 4(2), 1–7. https://doi.org/10.5815/ijieeb.2012.02.01
Rokach, L., & Maimon, O. (2015). Data Mining With Decision Tree (H. Bunke & P. Wang, Eds.; 2nd ed.). World Scientific Publishing Co. Pte. Ltd. http://www.worldscientific.com/series/smpai
Romero, C., Ventura, S., Pechenizkiy, M., & SJd Baker, R. (2011). Handbook of Educational Data Mining.
Salloum, S. A., Alshurideh, M., Elnagar, A., & Shaalan, K. (2020). Mining in Educational Data: Review and Future Directions. Advances in Intelligent Systems and Computing, 1153 AISC, 92–102. https://doi.org/10.1007/978-3-030-44289-7_9
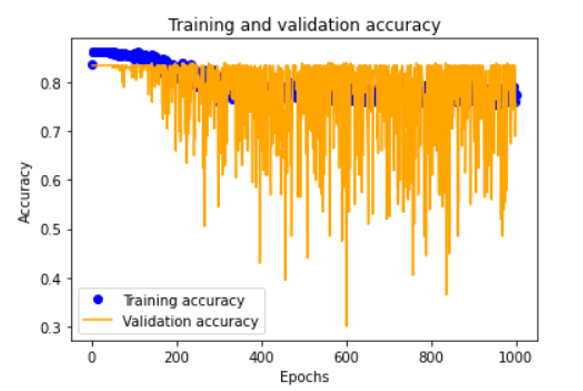
Downloads
Published
Issue
Section
License
Copyright (c) 2023 Uswatun Khasanah, Dwi Astuti

This work is licensed under a Creative Commons Attribution-ShareAlike 4.0 International License.
Authors who publish with this journal agree to the following terms:
- Authors retain copyright and grant the journal right of first publication with the work simultaneously licensed under Creative Commons Attribution License that allows others to share the work with an acknowledgement of the work's authorship and initial publication in this journal.
- Authors are able to enter into separate, additional contractual arrangements for the non-exclusive distribution of the journal's published version of the work (e.g., post it to an institutional repository or publish it in a book), with an acknowledgement of its initial publication in this journal.
- Authors are permitted and encouraged to post their work online (e.g., in institutional repositories or on their website) prior to and during the submission process, as it can lead to productive exchanges, as well as earlier and greater citation of published work (See The Effect of Open Access).